Data and AI have become an integral part of businesses today and are playing an increasingly critical role as an unparalleled force in shaping competitive advantage.
If data is the fuel of the digital economy, then AI is the new electricity.
To delve further into the captivating realm of AI and learn from tangible AI use cases that have driven business success, DigiconAsia sought out some perspectives from Armin Haller, Director, Data and AI Center of Excellence Head, APAC, Crayon.
How do you see Asia Pacific businesses unlocking new business opportunities and competitive advantages by harnessing the power of Data & AI?
Haller: Harnessing the power of Data & AI Solutions is not limited to the APAC region, but it is also happening globally. Although the emergence of ChatGPT and its capabilities has been at the forefront of conversations in the past six months, innovation in Data & AI has always been an ongoing process, and one such example is in the use of computer vision – which was mentioned in Gartner’s Hype cycle last year in the “Slope of Enlightenment Phase”.
During that point in time, computer vision was already in the productive stage, adding value for companies in use-cases such as face recognition for entrance into sports stadiums, or managing the quality of the products in a shop floor. Additionally, we have also seen it in driver assistance systems for mining companies, where the technology is used in ensuring that employees are not physically tired when driving heavy equipment that requires proper safety.
What we are seeing this year is the use of data models, AI models, or large language models (LLMs) being excellent in making sense of unstructured text – sometimes even better than a human being’s capability. This is hardly a surprise, considering that humans could not win against an AI in chess since Deep Blue was released in 1997.
We are currently in an era where AI models are better in understanding unstructured text in certain areas than we are as humans. For example, sentiment analysis has seen the potential of AI advancements with large language models – the likes of ChatGPT – being better at recognizing sentiment in text than we are.
Another evident use-case is in the ranking of resumes when a company is hiring for a position. Let us say a company has 500 applications and naturally, the employer would need to go through these applications looking at key factors such as experience or a certain skill. LLMs can filter out these applications in a time-efficient and accurate manner by ranking them according to some criteria set for the job.
Overall, this technology is transformational in many industries that requires the analyzing of text.
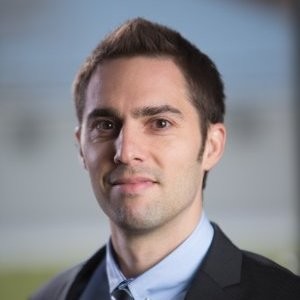
Please provide examples of enterprises adopting Data & AI solutions to create new revenue streams.
Haller: Enterprises across various industries have successfully adopted Data & AI solutions to create new revenue streams. Crayon’s center of excellence has been running for around 9 years and has undertaken around 300 use cases for different companies, adding value and generating new revenue opportunities across varying industries and verticals.
Prominently in the Manufacturing industry, there are plenty of use cases such as using AI to analyse health and safety records to ensure compliance and enhance workplace safety, using AI-powered visual quality inspection to maintain the high quality of products which would improve customer satisfaction by reducing defects, or even in high-end manufacturing processes such as air craft engines, in which AI will come into the fold to provide predictive maintenance, optimized performance, and reduced costs.
Use cases in other industries include the maritime industry, where AI can optimize ship scheduling and detect rust in ships, the agriculture industry, through leveraging drones or satellite imagery to monitor crops for improved yield and better resource management, and even invoice processing where companies can process thousands or millions of invoices annually.
In the content creation industry, both content creators and marketers alike are using the power of AI to leverage Chat GPT using other tools such as Midjourney that can generate imagery as well as LLMs that can efficiently output video summarization.
Software engineering can also be accelerated with programmers being able to dedicate more time on value-add activities rather than rewriting a specific for loop or a function that has been executed a million times before.
What are some obstacles organizations faces when implementing and integrating AI solutions into their business operations?
Haller: Naturally, the first obstacle of any emerging technology is the lack of resources and the lack of skill – either within the company or globally on the marketplace of people who have the skillset to implement these solutions.
A new job title in demand for the technology industry is for an LLM engineer, who is a combination of a data scientist and a machine learning engineer specifically trained to tune and improve the quotation mark to improve LLMs – like how there are parameters for ChatGPT, and users get a better answer through a more structured way.
The next challenge would be the barrier of entry to access these LLMs although many of these models are open source. However, many people do not have access to these open course models, with companies like AWS or Microsoft allowing other companies to use this technology through a serverless AI model – which can be taken off the shelf and applied to a problem scenario. Professional service companies provide the models that has already been trained – which is the extremely costly phase.
How can they overcome these challenges?
Haller: As many organizations may not have the in-house expertise to handle AI implementations, collaborating with professional service companies that specialize in AI can provide access to skilled LLM engineers, ML engineers, solution architects, and data scientists. These service providers can augment the company with the necessary skills and ensure the implementation is carried out effectively.
Organizations can also explore SaaS solutions with a company that provides AI model integration that aligns with their specific needs, such as scheduling, invoice processing, legal document interpretation, and more. This saves time and effort, as these solutions are pre-built and can be easily adopted to address common business challenges.
Some organizations may have highly specific requirements – such as aircraft manufacturers – that cannot be addressed by off-the-shelf AI solutions; however, they can invest in building custom AI models that are tailored to their unique processes and operations.
Companies can invest in building managed services that focus on AI-driven solutions for specific business tasks, such as invoice processing or legal document management. These managed services can be offered to other businesses, generating new revenue streams, and sharing AI expertise across industries.
The implementation of AI within an organization’s operations should be a gradual journey, and these organizations can start identifying specific use cases with clear ROI potential, before expanding their adoption across other different areas of the business.
As AI often involves processing sensitive data, organizations must prioritise data privacy and compliance, this can be resolved through Implementing robust data protection measures and ensuring adherence to relevant regulations build trust and credibility in AI adoption.