The following approach to data classification may be self-evident, but in practice, even minor deviations or shortfalls may lead to challenges.
As data continues to grow in volume and importance, South-east Asian organizations will increasingly need a well-honed classification strategy for processing their data and for making informed decisions about storing, accessing and sharing information.
All data is not created equal — and is not equally valuable. By classifying data based on attributes such as sensitivity, value, and regulatory requirements, organizations can establish clear and efficient guidelines for how long they should retain and store each data category.
For instance, sensitive customer information may require extended retention periods due to legal obligations, while non-critical operational data might need shorter retention periods.
Ultimately, good data classification enables organizations to reduce storage costs, minimize clutter and tighten adherence to compliance regulations. As a reference, the ASEAN Data Management Framework stipulates in its guidelines that data management policies should provide clarity to internal and external stakeholders on the ways in which the organization handles data.
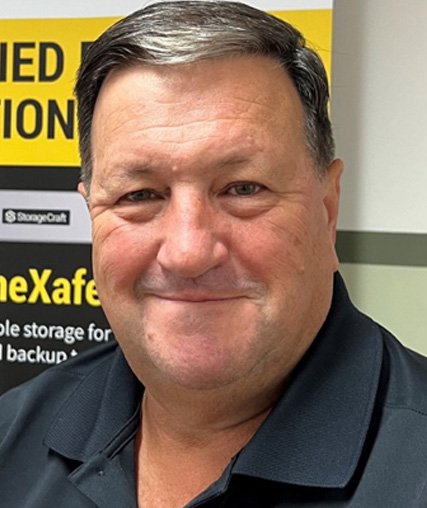
Consequences of poor data classification
Not having a solid data classification system exposes organizations to significant risks.
- When they cannot differentiate between critical and non-critical data, their storage resources can be overloaded with redundant or outdated information, resulting in unnecessary costs.
- Additionally, organizations may struggle to identify data subject to retention requirements without proper classification, which may lead to non-compliance and therefore, legal repercussions.
- Furthermore, poor data classification system hampers security. Without clear guidelines on data handling, employees may inadvertently mishandle sensitive information, neglect encryption protocols, or share it with unauthorized recipients and store it on insecure devices. All these constitute cybersecurity and data protection risks.
One major obstacle to establishing solid data classification is the sheer volume and diversity of data generated across different departments and systems. Additionally, data may be stored in various formats, file types, and locations, further complicating the process and possibly leading to misclassification.
Finally, a lack of employee awareness and training about the importance of data classification can also hinder adoption.
Four steps to solid data classification
Here is a data classification navigational guide that will lead to easier access, enhanced security, and improved decision-making:
- Establish a cross-functional team
A taskforce involving IT, data management, legal and compliance experts to define clear classification criteria can ensure the classification system aligns with regulatory requirements and business goals. - Invest in automation
Data classification tools and software that automate the process can scan and analyze data to assign appropriate labels or tags based on predefined rules. This automation offers multiple advantages, including dramatically reducing the potential for human error, a common problem in manual classification efforts. The result will be a higher degree of accuracy in data classification and a reduced likelihood of sensitive information being mislabelled or improperly handled. Finally, automation ensures a consistent classification standard across diverse data sources and types, eliminating discrepancies that could arise from variations in human interpretation or judgment. - Boost training and awareness
Organizations should educate employees about the significance of data classification, the potential risks of mishandling data, and the proper procedures to classify data accurately. This education helps foster a culture of data responsibility throughout the organization. - Keep your strategy dynamic
Data classification is more than just a one-and-done exercise. It is an ongoing task that demands continuous monitoring and adjustment. The world does not remain static, nor does your data. Proper classification requires periodic reviews and updates to accommodate changes in data types, regulatory requirements, and business needs. Regular audits and assessments can identify areas where classification may have deviated from standards, or when new data categories have emerged.
A good classification strategy empowers organizations to harness the true potential of their disparate data, and turn chaos into clarity.