In the accelerating digital age, whichever financial service innovates fastest with data captures the most opportunities. Here is why …
In the financial services industry, data paves the way for every strategic move. Whether it is to create a new service, comply with regulations, or overhaul and re-engineer legacy operations, central to the effort is data.
The pace at which organizations providing such services can reshape and repurpose data is key to determining their ability to predict market trends and meet client expectations accurately.
For the established players, the main issue is that they are still taking too long to get the data they need to support the development of advanced analytics, AI and machine learning (ML) projects or research. Ultimately, this lag time slows the development of innovative new products and restricts the growth of these institutions.
The most effective solution is to immediately, if not gradually, move toward creating an agile data infrastructure. Sometimes called a ‘data fabric’, this approach affordably supports creating and maintaining the huge number of data pipelines needed to compete in today’s financial services marketplace.
Real-time everything
The twin demands for instantaneous knowledge and the anticipation of constant change are what driven the financial services industry toward agile data.
Hyper-personalization, data-led listening and ‘real-time everything’ are now the norm. The standard against which financial services are being judged nowadays has been set by digital consumer businesses and fintechs that are digital natives and innovating in the latest services such as neobanks.
Whether a person is making a payment, sending money across borders, or anticipating market data for an investment decision, anything slower than “right now” would cause perceived inconvenience. ‘Slow’ processes are the visible reflections of cumbersome systems that are incapable of meeting modern requirements.
Can DataOps help?
While financial services firms are masters of data capture, creation and storage, capturing customer details, processing transactions and keeping records of accounts, their data platform may not have been built for a real-time world that feeds huge rivers of data to analytics, AI, or ML apps.
Their existing legacy data infrastructure is either static and/or fragmented across data silos or stuck on legacy platforms incompatible with today’s requirements, thereby creating mismatches between products and underlying data that extend to a rift between expectations and deliverables.
This is where DevOps and DataOps come in, offering real-time delivery and rapid change. Such organizations can adopt an agile data platform to supports DataOps and enable rapid change and stable operations.
DataOps is an approach to data analytics that deploys automated, process-oriented methodology to improve the quality of, and reduce the cycle time of, data analytics. It is how organizations can manage every step—from the source of the data source to the consumer of that data—in a manner that improves efficiency, ensures data security, and reduces costs.
Like the now-common DevOps for software development, DataOps combines agile development with operations management for data. The methodology of an agile data platform is built on three layers:
- Agile data flows
These enable the operationalization of analytical and ML models without significant development while maintaining the agility of data pipelines. They move the organization away from static, batched and siloed data pipelines to allow data to flow freely through the organization. - Converged analytics and machine learning
Operationalizing AI, or AIOps, creates a powerful, unified, easy-to-use data modeling and analytical environment. This environment can be deployed in a wide range of scenarios, including quantitative research, business analyst functions and operations activities, enabling data to drive agility at all levels within the organization. - High-speed data fabric
To allow raw data to be ingested and passed directly to analytic data engines and ML functions in near-real-time, transitioning from data warehouses and data lakes to hybrid data architectures that are able to leverage high-speed data substrates is required. An effective data fabric is important for managing the distributed environments of an enterprise, ecosystem and supporting navigation, from the edge to the core, to the Cloud.
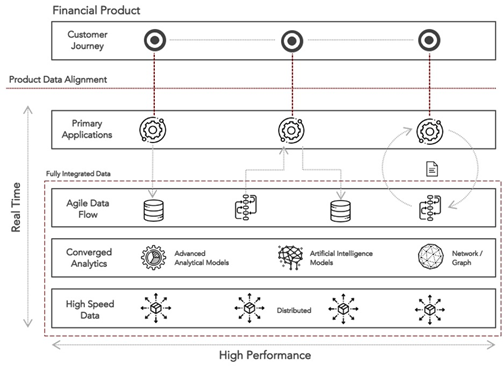
In the figure shown here, for organizations to remain competitive, the minimum effort required would be to adopt the foundational layers of fully integrated data.
Thriving in the digital age
Agile Data Platforms power modern experiences and meet business requirements. Some use cases that reflect both new and enhanced products, as well as modernizations to operations, could involve:
- Enabling more accurate credit decisions by incorporating advanced ML algorithms across very large datasets in near-real-time
- Automation of mass wealth client advisory and investment planning that tailors advice and automates custom portfolio construction and management activities
- Development and maintenance of ML training pipelines, ensuring that models are updated with the latest data and are accurate and auditable
- Providing a high-performance computing data platform for computationally-intensive risk calculations and trading models
- Analyzing customer interactions, creating intelligent products and customer journeys that can be optimized in real-time based on streaming interaction data
By virtue of the rising costs incurred to support bespoke solutions on legacy infrastructure, as well as the pain of adapting data to meet the needs of new applications, financial services firms can consider implementing agile data platforms based on DataOps to accelerate innovation and competitiveness.