Having the right digital culture in place determines how successful an organization can be in their data and AI strategies.
Data-driven strategies have become the ‘north star’ for enterprises, in hopes of utilizing their existing databases and customer insights to improve efficiencies and better inform business decisions.
With generative AI making a splash in the tech scene, the need for organizations to step up their data strategies – with data quality essentially shaping the success or failure of any AI venture – has become more imperative than ever.
However, companies often see a specific technology as the single solution to reaching this goal, when culture – and the right processes in place to remove data silos and encourage collaboration/data sharing securely – is what truly makes or breaks this transition.
While culture cannot change overnight, DigiconAsia finds out from Nicolas Sekkaki, Group Practice Leader for Applications, Data, and AI, Kyndryl what are the changes that companies need to make today – and steps they should take – to not only supercharge technology innovation but ensure its sustainability and effectiveness in the long run.
What are some barriers that prevent a digital culture from evolving alongside technological innovation among Asia Pacific organizations?
Sekkaki: It has become increasingly clear that it is not enough that organizations view information as power. There needs to be a cultural shift and collective understanding that data can only reach its true potential –– transform into knowledge – when shared and embraced across the board. While 73% of CEOs surveyed by Frost & Sullivan agree that deriving value from data has become a top business priority, there remains an evident disconnect between organizational goals and actual business results.
According to a Kyndryl-commissioned research with analyst firm Ecosystm, while organizations in ASEAN will increase data and AI usage across businesses in the next two years, close to half (48%) remain challenged in building scalable solutions due to lack of understanding by end-users. And only 7% of these organizations prioritize building the right data and AI foundation.
The key barriers that we are seeing that affect companies’ data culture include:
- Misalignment of data strategies and business goals: Different stakeholders may focus on individual components of data, be it protection or visualization, but this rarely finds itself integrated with wider business goals. This disconnect creates fragmented strategies that cannot truly support basic functions, let alone drive profits at the right time.
- Shortage of talent & skillsets: Few companies have the dedicated expertise to leverage the value of data across an organization. Firms often think that a single team or one-time expenditure on a new software can make waves of change within its data network. However, the continued evolution of data requires skills to be upgraded in tandem. The lack of continued investment in data literacy has made the complexity of streamlining data even more daunting, with the workforce unsure of where they can find data, or what they can do with available data to supercharge innovations in AI and ML.
- Eroding trust in data initiatives: If data, and the skills needed to understand it, is not thoroughly embedded across every function, change-management processes can face roadblocks with more teams losing sight of data-led strategies. These can be further exacerbated by heightened pressures to produce results from patchwork strategies.
There is no denying the power a strong data culture has on enabling digital transformation and innovation. Great digital culture, in turn, needs to be enabled by the right people, integrated workflows, and a collective understanding of what data they have, how they can access it, and what they would like to see it produce.
How is the quality of data limited by under-developed cultural processes? How could organizations remove these cultural barriers?
Sekkaki: Data is a tool that will always fall short of its potential without the right teams or people behind to direct its use. Culture can thus make or break the quality of data and, in turn, the insights and innovations, including AI, that it ultimately fuels.
One of the most overlooked problems of an under-developed data culture, is the failure to cultivate and integrate a data strategy that truly aligns with business goals. From the get-go, this puts important processes like data governance on the back burner. Without the standard processes and ownership of how to handle, define, and interpret existing data, organizations will find themselves buried under a deluge of “bad data”, and be inundated with unreliable information that will have a palpable impact on day-to-day operations and future business decisions.
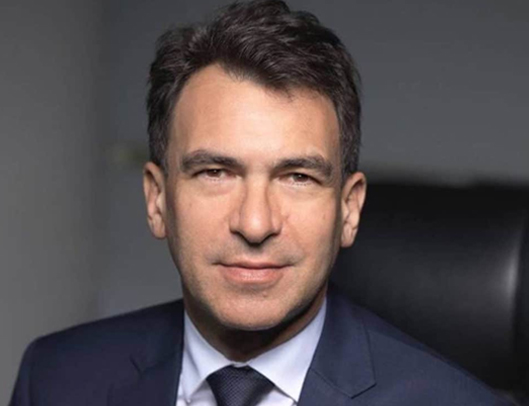
The lack of communication about data between cross-functions also fuels the development of silos. This creates a disjointed data experience, with employees who need data the most, finding it difficult to access and make use of information across multiple touchpoints, fueling inaccuracies that fail to reflect where the organization truly stands.
There are concrete step-changes that organizations can take to accelerate the development of stronger data processes and the breakdown of data silos:
- Alignment on data use across functions: Chief Data Officers need to be a part of the C-level that is directly accountable to the business results of the organization. This empowers them to take bold steps forward, including moving away from legacy systems and driving data literacy campaigns extending from the board room to the employees, ensuring a collective understanding of data governance policies. This will improve communication about data and remove silos.
- Investments in skillsets and tools: True data culture cannot rest with a single team. As technologies evolve, so must data, and the ways we treat it. This means committing to ongoing investments in systems and people, ensuring that they are kept up to date with recent developments in the sector. This weight could be shared with trusted partners who are able to offer the expertise to make these solutions more accessible.
- Continuous evaluation of data strategies: The value of data, and investments in technologies dependent on data, will take time. To ensure its relevance, and to uphold the teams’ trust in data solutions, businesses need to continuously reflect and update processes according to evolving situations, goals and KPIs.
Would you agree that data is the true enabler of AI? If so, how should enterprises utilize existing data resources/infrastructures to generate concrete results from their AI investments?
Sekkaki: Yes, because for a machine, like AI, it can only be as reliable as the data that it receives. And, for AI to be successfully scaled it must have a consistent source of data, for it to be fed and learn from. However, a study from S&P Global Market Intelligence uncovered that 65% of enterprise respondents still have difficulties accessing the required data to build and train their AI models. These challenges reflected the gaps present today, that companies are aware of and collecting on massive amounts of data yet are not implementing the right architectures needed to streamline and share data.
Culture is key to bridging these foundational gaps. Some immediate changes include establishing clear business reasons for investing in data and AI systems, aligning your data strategy and all relevant stakeholders to your business goals, ensuring that there is collaboration across all teams – data science, functional etc. – and driving the necessary data literacy programs to grow your collective understanding.
Once a strong data culture is established, it is important to ensure that data can be accessed by the stakeholders who need to leverage it real time. The right data foundation is critical to make this a reality. Implementing a robust, agile data strategy that ensures not only real-time access to clean and trusted data, but also data identification and interoperability, will unlock the potential of enterprise data.
Another reality to be mindful of, is the prolonged shortage of technical skills and expertise. In addition to driving programs that continuously upgrade skills, partnerships with third-party solution providers have proven to be increasingly key to ensuring access to the latest insights and best practices of how to manage data and maximize success of these projects.
As more companies integrate AI into their processes, what are some key steps that should be actively implemented to ensure ethical and reliable use of AI?
Sekkaki: The outcomes that AI can enable means the onus now is on companies to quickly embrace such technology to create better experiences for customers and the wider public.
However, before AI becomes pervasive – it must be ethical, explainable, and sustainable. To this end, organizations must continue to correct or remove biases from its AI models, understand the reasons behind its results, and train their systems more efficiently.
Since the data a machine is fed directly impacts the results, businesses need to first prioritize the quality and organization of their data before they begin implementing AI at scale. This starts with a thorough discovery phase before embarking on any kind of data transformation initiative.
Additionally, data governance must also become increasingly prioritized to pave the way for successful and ethical AI initiatives. Such structured processes are critical in enabling better decision-making, improving trust in data, protecting data, and ensuring data consistency. Effective data governance can also provide organizations with insights that can lead to tangible benefits such as driving revenue growth and managing data privacy and risk.
How should business and technology leaders in an organization tackle the challenges and inertia toward AI within their corporate culture?
Sekkaki: Companies need to understand that truly enabling AI for the business will take time, investment, and commitment from leaders across an organization. Such efforts should stem from first looking towards data as a foundational resource.
To enable AI at scale and overcome existing cultural inertia, companies must first have a solid data foundation that creates a strong and agile data strategy.
Ultimately, data-driven organizations that embrace emerging technologies and identify opportunities to infuse AI into their operations will be well-positioned to navigate the competitive landscape.