With the right reporting frameworks and AI-driven compliance validation tools, investors can evaluate potential partners’ ESG progress accurately and transparently
The impacts of climate change have given rise to environmentally conscious consumers and investors, and governmental regulations on carbon emissions. With pressures from these groups, corporations can no longer take their environmental, social and corporate governance (ESG) and sustainability goals lightly.
However, according to some studies, close to 80% of these responsible corporations are pursuing their ESG goals without a proper corporate sustainability strategy. A lack of the right skills, knowledge, and data to support these initiatives hampers their efforts.
Hence, there is a need to examine the challenges that businesses face in meeting these goals, and how they can exploit technology to gain clarity from and with ESG data, in order to be empowered to reach their goals in the best way.
Substantiating and seeing-through ESG data
With so much attention placed on ESG, many corporations are already publishing their ESG-related data in their annual reports even though it is not compulsory. Yet, despite this voluntary reporting, there is no clear way to substantiate the claimed data and progress, to gauge if a corporation is really any closer to achieving sustainability goals.
To bridge and standardize this data gap, reporting frameworks such as the Sustainability Accounting Standards Board (SASB), the Global Reporting Initiative (GRI), and the Task Force on Climate-related Financial Disclosures (TCFD), are being developed. However, due to the complexity and scope of ESG, it can be challenging to evaluate an organization’s or investment’s ESG accreditations using a single reporting framework.
Therefore, corporations must:
- appraise both their internal structure and their wider supply chain networks
- ascertain that the suppliers they work with are also ESG compliant — a process that is increasingly done using data from the supply chain and external sources to verify and evaluate compliance
It is critical to emphasize the importance of accurately understanding the data, as there is the issue of firms giving the impression that they are more ESG compliant than they are in reality, which can lead to allegations of greenwashing.
Natural language processing can help
Fortunately, advancements in AI can assist organizations in gaining better and accurate insights from their data. For instance, Natural Language Processing (NLP) is capable of capturing and arranging ESG concepts with both positive and negative sentiments within the data.
This technique makes use of Name Entity Recognition (NER), which can identify and categorize unstructured textual material. It is more than just an ordinary matching mechanism that matches words based on a pattern.
In order for NER to function, named entities that are present in unstructured text must be located, identified, and mapped into pre-trained ESG categories. For instance, environmental classifications such as emission reductions, net-zero commitments, and the use of green energy, can all be identified.
Additionally, compute-intensive AI and NLP tasks can be accelerated through the use of high-performance data infrastructure that makes use of both specialized computers and networks. These technologies allow for the creation of data pipelines for the accelerated training of NLP models.
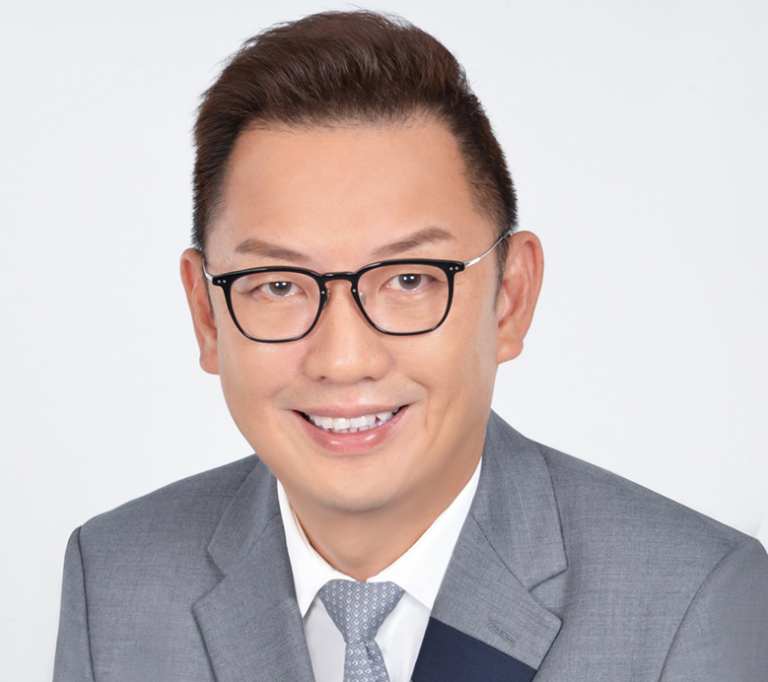
Numerous ESG reporting and compliance frameworks, as well as particular corporate reporting methodologies, are evolving. Placing the different frameworks on a graph structure, one commonality emerges: they can be used to assess ESG investments and corporate supply chains:
- Utilizing an ESG knowledge graph and pre-classified NER, data can be mapped against the structure of a graph to create a unified view of an investment or company’s ESG status using both historical and real-time data
- After the graph has been filled with verified data, analysis may be conducted to produce dashboards, reports, and ad-hoc analytics. In addition, graphs offer sophisticated analytical capabilities that open up fresh perspectives on data and facilitate deep comprehension of an investment or company’s ESG compliance action
- Graph algorithms can be used to analyze the structure and find new links and emerging patterns such as negative sentiments and groupings around particular ESG issues
- By combining reporting frameworks and graph topologies, corporations can create and substantiate complex ESG reporting, disclosures, and underlying unstructured textual data
Gaining these insights, corporations can then present their findings to investors and supply chain partners, providing accountable assurance to potential investors and partners that their ESG claims are founded on facts and trustworthy data.
Gauging the data before committing
Similarly for potential investors and partners, AI tools can be used to validate ESG data offered by the firm they are interested in. It is possible to train these algorithms to analyze the tone of the narrative by matching the words used with a taxonomy of reference words and concepts. The algorithms can also be trained to identify ESG topics in unstructured data.
For instance, transcripts of a firm’s quarterly earnings call could be examined by NLP to determine the tone of the words used in a senior representative’s pitch. This would quickly identify which sections of the presentation cover ESG topics, and sentiment-analysis of the words can be used to make a high-level evaluation of a firm’s real commitment to achieving sustainability goals.
For potential business partnerships, even with due diligence procedures and spot checks, firms may still encounter difficulties in accurately identifying ESG compliance, due to the complexity, number of suppliers, and length of chains with primary and subcontracted suppliers.
To ascertain whether suppliers have adhered to their ESG contractual responsibilities, it is essential to be able to evaluate supply chain data from internal and external data sources. Again, with the right technologies at hand, corporations can examine textual data, as well as real-time data from satellites or ground-based sensors, to monitor greenhouse gas emissions or pollution from manufacturing processes. The resultant information, when analyzed and processed in graphical form to present clear, transparent and easy-to-verify ESG claims, will be useful for all parties concerned.