This writer recaps the pros and cons of generative AI while reminding us the as-yet-indeterminate pitfalls of its misguided/irresponsible use
The hype surrounding generative AI remains palpable in 2024, with businesses of all sizes rushing to harness its transformative potential.
The reality is that for years now, some organizations had already been using Large Language models (LLMs) to unlock tangible benefits.
The enterprise generative AI (GenAI) revolution, however, is just getting started, and we will see greater innovations in AI and ML technologies that will bring about more business value, and tools that redefine the way we work.
Cutting-edge potential
Looking ahead, we can expect more organizations to leverage GenAI for various language and image-related tasks, including natural language generation, document understanding, content search, summarization, and augmentation.
These new cutting-edge capabilities will not only allow businesses to unlock increased productivity through streamlined tasks and processes, but they will also increase overall efficiency and influence smarter decision-making. For example, organizations will be able to leverage GenAI capabilities that empower finance teams to streamline collections from customers and recapture missing funds sooner.
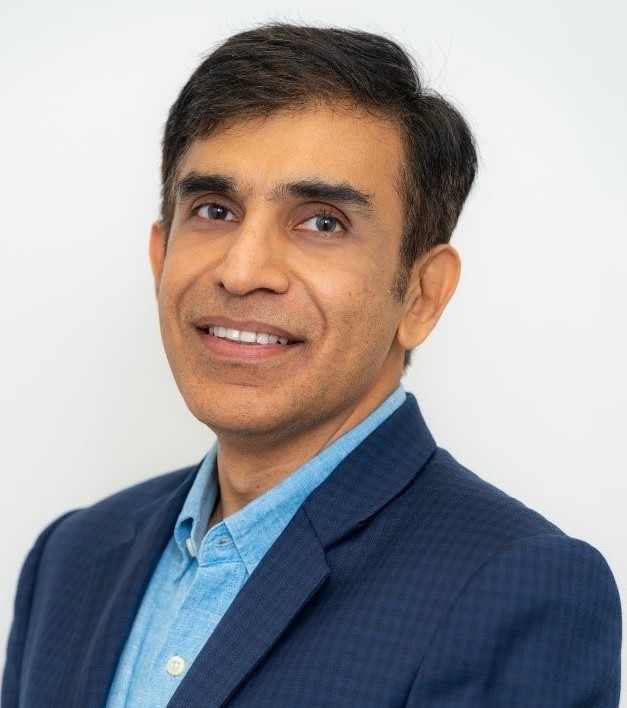
Finance teams will be able to automate the process of crafting past-due notices with recommendations on the tone of the correspondence, driven by how late the customer is, or how often they are late, to save valuable time. Finance teams will also be able to automate letters in bulk, based on configured rules, to easily deliver an entire package, including past invoices — enabling collection agents to greatly increase their throughput and recapture missing funds sooner.
Meanwhile, human resources departments will also be able to leverage GenAI capabilities that enable hiring managers and recruiters to generate job descriptions in minutes versus hours. By leveraging information already stored in one platform that acts as the single source of truth for people data, hiring managers can create precisely targeted job descriptions to solicit the best candidates.
Harnessing GenAI without the quirks
It is important for organizations to note that the effectiveness of GenAI hinges upon not just the quantity of data, but the quality of the data used for training. As evidenced by the many stories highlighting how GenAI technologies produce confabulations, LLMs are only as good as the data that feeds them.
The foundational models that have dominated headlines have been deliberately trained to solve a broad class of problems from the broadest set of data available. That vast set of data is not all of equal quality or of well understood provenance. Unsurprisingly, this can result in erratic, incorrect, unsafe behavior, or infringement of intellectual property.
There have also been instances where the safeguards on responses put in place to deal with poor training data have not held up over time. Research has shown that machine learning models benefit from the simplicity and consistency of data inputs. To address this, AI systems need to focus on targeted, domain-specific models and high data quality above all else, to provide outputs businesses have confidence in.
One way to increase the possibility of GenAI consistently generating accurate, meaningful, and trustworthy results is to ensure the use of massive, high quality datasets.
Implementing GenAI responsibly
While the promise of AI is undoubtedly thrilling, it is also important to acknowledge that its use can pose some risk. Upholding data security and privacy standards should be of utmost importance when deploying AI. With a stringent AI ethics framework, businesses can appropriately manage risks without sacrificing any of the benefits that innovation brings.
Even though AI provides ample opportunity to automate business processes, the emphasis should remain on augmenting and not displacing people. Machine learning and deep learning models should be used to elevate the human potential rather than replace them. For example, across people management functions, the application of AI would focus on developing job-relevant skills solutions that enable more personalized experiences, nurture career development and growth, and promote internal mobility.
AI has the power to foster collaboration between humans and machines, and be designed to integrate human expertise. To this end, a “human-in-the-loop” approach elevates AI-generated recommendations and decisions.
As we navigate the ever-evolving AI landscape, there needs to be a balance between innovation and responsibility, so we can shape a future where AI benefits society. Only then can we ensure that hype does not overshadow practicality, and that the workforce is empowered to thrive through various innovations.