Get your ‘data house’ in order before leveraging the potential of generative AI for your business.
As data underpins all AI capabilities, it is vital that companies use the right data in embracing generative AI’s potential to transform many industries. In fact, McKinsey has found that a modern data and tech stack is a key strategy for a successful approach to generative AI.
Qlik introduced in June 2023 a suite of OpenAI connectors that will help users seamlessly and securely bring generative AI content into Qlik to support a wide range of cloud analytics and automation use cases, and more recently Qlik Staige for a trusted data foundation, automation and actionable predictions to make AI real with organization-wide impact.
Qlik’s acquisition of Talend is a clear indicator of its commitment to help organizations get their ‘data house’ in order – in the most efficient way possible. DigiconAsia finds out more from James Fisher, Chief Strategy Officer, Qlik.
What are some concerns and challenges that organizations face when implementing new capabilities like generative AI?
Fisher: While we see excitement about generative AI’s transformative potential, many of the applications are still to be fully defined and understood. This causes fear and uncertainty as companies are not yet sure of how to best leverage the technology. These companies are pulled between acting quickly to avoid being left behind and proceeding with caution to avoid major missteps.
Bridging the trust gap will take time as more users understand the technology and how it can help with sound decision-making processes. This is a natural reaction whenever a new technology comes to the market.
For example, during the advent of the internet in the early ‘90s, there was a lot we didn’t know, like whether providing unlimited access to employees or students was a good idea. Similarly, people today are skeptical about letting their teams use generative AI to make decisions as businesses are still largely led by teams who want human beings to do the work.
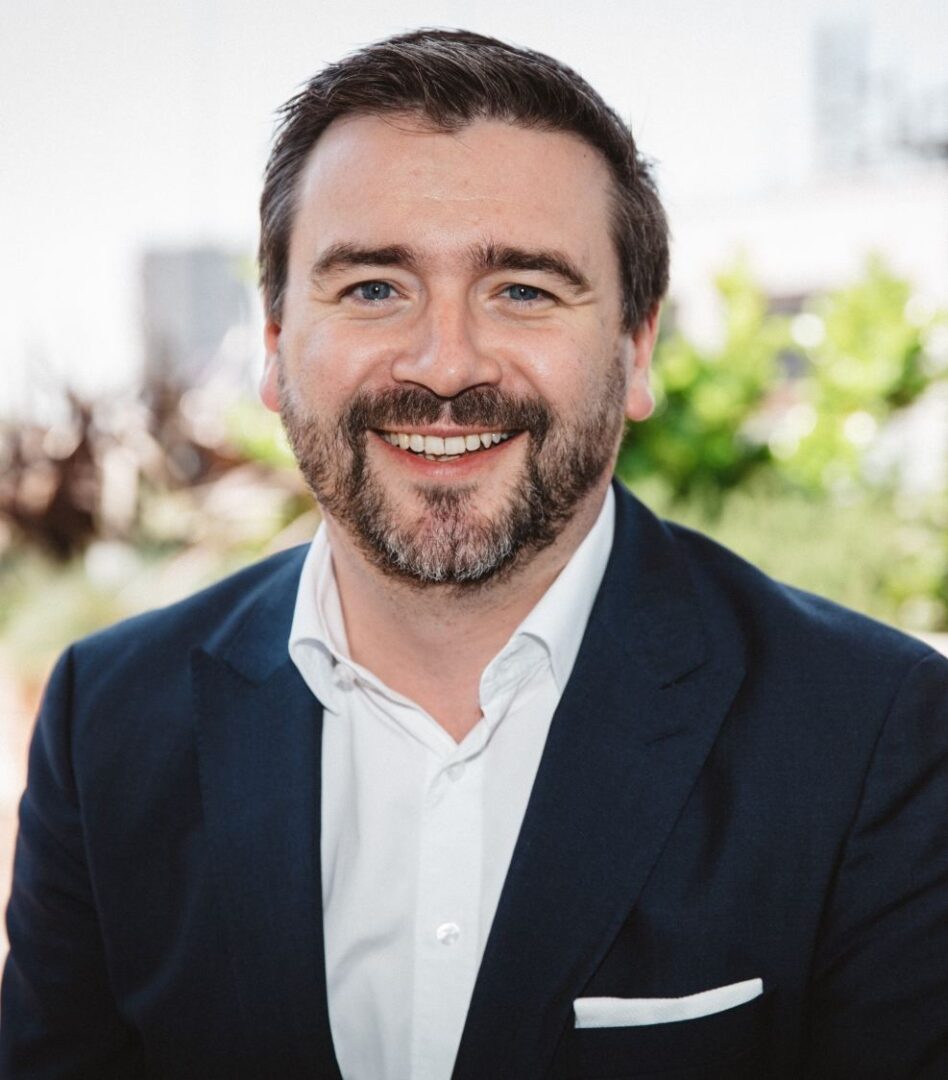
As generative AI capabilities are underpinned by large volumes of training data to learn patterns, relationships, and structures in the data, one of the major concerns is ensuring high data accuracy while preserving security, privacy, and governance.
Without management, tools, and frameworks in place, organizations face the challenge of mitigating the risk of false conclusions based on inaccurate or incomplete information or the possibility of biases sneaking into AI model results.
What data considerations are critical for adopting generative AI for business? How does effective data management and governance form the bedrock of working successfully with generative AI?
Fisher: Chat-based generative AI starts with a prompt that lets users submit a question along with any relevant data to guide the content generation. Developing precise questions and having accurate data are essential for the effective use of these tools as the quality of the responses is directly tied to the quality of the questions presented.
Data must be organized and trusted when using it as a source for generative AI, as this information will become training data that may eventually re-surface at a later stage in response to someone else’s question. Combining data from different sources and in various formats is critical for effective data management and governance as this enhances the overall quality and robustness of AI algorithms while mitigating biases and limitations that may arise from relying on a single dataset.
Importantly, respecting data privacy and learning how to tackle any potential biases ethically are critical to working with generative AI successfully. To achieve this, organizations must comply with data security and compliance regulations and processes. Any poor quality or uncontrolled data can compromise an organization’s ability to use generative AI to make smart business decisions, build great digital experiences, improve process efficiency, or even encourage innovation.
How is Qlik working with customers to help them harness the capabilities of data and generative AI to tackle business challenges and improve outcomes? Please share some examples.
Fisher: Today, AI is infused through Qlik’s products via our Insight Advisor technology, which allows customers to ask questions in natural language to uncover insights, suggest the right way to visualize data and to create complex analysis. We also added a low-code machine learning capability which allows customers to easily create ML experiments, identify key drivers in their data and train models to not only see what might happen but why.
Qlik works with AusCycling, Australia’s national sporting organization for all forms of cycling, to improve operational processes and efficiencies. By using QlikCloud, which incorporates these AI and ML capabilities to help users understand and use data more effectively while minimizing cognitive bias, AusCycling successfully eliminated membership data access and reporting issues by bringing together all the data into one platform.
In a bid to move the organization towards a data-driven culture, we are helping AusCycling get to know their customers better, harness their product and membership usage to personalize engagement, and build differentiated products.
We also work with NZ Health Partnerships (Hono Oranga Aotearoa), part of Health New Zealand (Te Whatu Ora), to support them with data verification and analytics for the Spend Data Repository, a central data repository of actual spending on medical devices. The organization was tasked with taking a national view of health spend data, starting with medical devices.
This information is held in the nine different Enterprise Resource Planning (ERP) systems used across the country’s 20 hospital districts. As the organization needed to produce regular national reports, it was challenging for the team before using Qlik as the data sat in different sources and formats.
By using QlikCloud, NZ Health Partnerships can now integrate its data into one single source of truth, easily share it with other entities without compromising accuracy and reliability while developing reports faster. The organization can also enable agile and flexible financial forecasting as well as help users widen their use of data and accelerate innovation across the sector.