What are the hottest topics in the cyberworld, and how would these technologies impact our world?
From chatbots to AI-powered call centers in customer service, from video game simulation to art and design in creativity, from autonomous vehicles to 3D modelling in smart cities, and from medical imaging to digital biology in healthcare – the applications of AI are deep and broad.
AI is also intricately a part of our future – in a world of digital twins and the metaverse.
DigiconAsia discusses with Tomasz Bednarz, Director of Strategic Researcher Engagement, APAC & EMEA, NVIDIA, developments in generative AI, deep learning, simulation and digital twins – and what may be in store for us in the industrial metaverse.
With the recent launches and public interest in generative AI, what do you see as the business opportunities and challenges that generative AI opens up?
Bednarz: There are many potential applications for this powerful technology, including in these areas:
- Text generation: question answering; creation of stories, marketing content, lyrics and even poetry.
- Customer service: chatbots; automated or AI-powered call centers and quick-service restaurants.
- Image creation: avatars, photos, paintings, story illustrations, 3D renders, marketing content.
- Programming: code generation; assistance for developers in identifying bugs and possible solutions.
- Business: synthesis or creation of new documents.
- Video: character animation; creation of scenes for social media; storyboarding.
- Simulation: generation and rendering of characters, objects and scenes in video games and virtual worlds.
- Autonomous cars, robots: creation of specific conditions for training, such as with data for environments with rain, snow, low light and other unusual or unexpected circumstances.
- Healthcare, medical imaging: drug discovery; synthetic training data for rare pathologies.
- Digital biology and protein synthesis: creation of new proteins to fight disease.
- 3D and digital twins: real-world capture in 3D; rapid 3D reconstruction; text-to-3D models generation.
- Art and design: enhanced AI-supported art concepts and design prototyping; generative geometry and architecture.
Some of the challenges that generative AI opens up include:
- Ethics: Engineering for building safe and trustworthy AI systems.
- Accuracy and trust: People must be able to trust the outputs of generative AI systems. These systems are still in their infancy, but will improve over time and provide results with greater accuracy. Current research focuses on such improvements.
- IP: There are important considerations that need to be debated and worked out. Artists who wish to protect their intellectual property should have the opportunity to opt out to exclude their work. AI systems should respect requests to exclude artists’ works if flagged as “not for model training.” There is opportunity for generative AI models to offer new IP licensing considerations.
- Computational resources: As the size of data grows, there will be increased need for larger computational resources, both to train the model and to generate outputs. For example, OpenAI used more than 10,000 NVIDIA GPUs to train GPT-3 models. New, accessible models will need to be established to enable the wider community to play a role in this AI evolution.
How do you see digital twins evolving, with advancements in AI, especially in terms of a more widespread application of digital twins?
Bednarz: We now have the technology to create true-to-reality digital twins of the physical world. This new evolution of the web will be much larger than the physical world because, as with the web, almost every industry will benefit from participating and hosting virtual worlds. Creators will make more assets for virtual worlds than for the physical world, and enterprises will build countless digital twins of products, environments and spaces — from object scale to planetary scale.
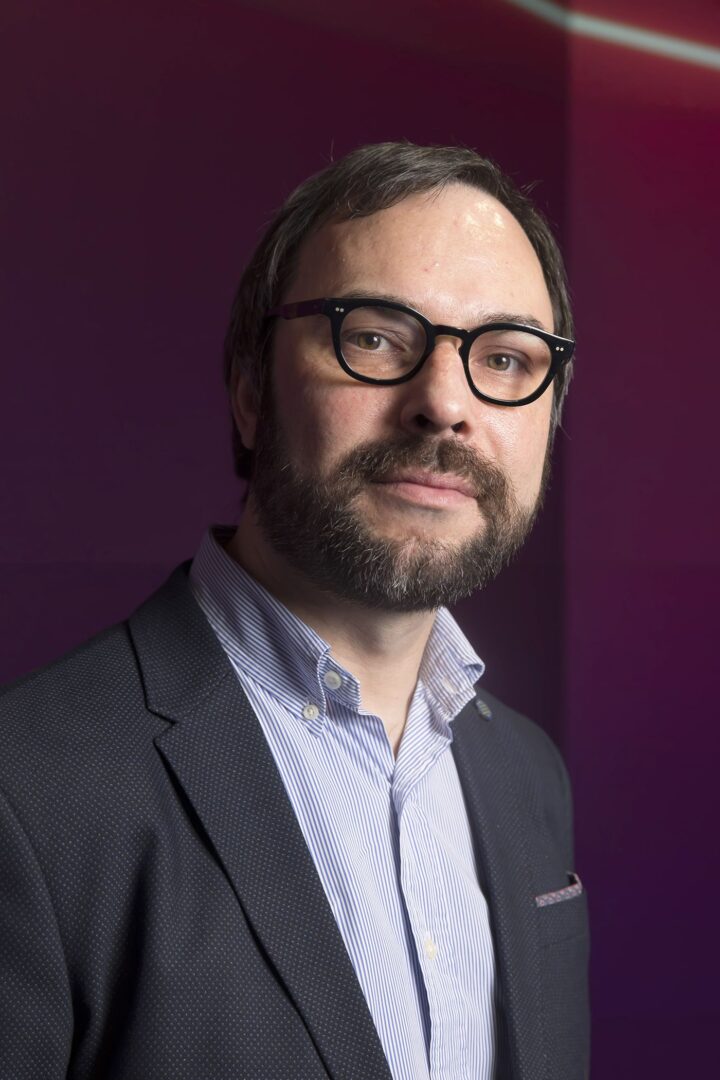
Simulation brings enormous opportunities for all enterprises, as simulating projects virtually before producing in reality will save costs, reduce waste, and increase operational efficiency and accuracy. NVIDIA Omniverse is a technology platform that enables connecting and building physically accurate virtual worlds, or digital twins, to help solve the world’s hardest engineering and science problems. Digital twins, with the help of AI and learning models, allow for these situations to be simulated in increasingly complex variations, reducing risks to end users, supporting more collaborative work and enabling formerly sequential steps to be parallelized. Data interoperability and creation platforms that can access these streams are a key requirement and will even offer the chance to design, test and operate new products entirely as live digital twins, from inception to physical replication.AI has also started to help capture the real, physical world in digital form through 3D models, point clouds, textures and more. The transfer of data from the real world to the digital world is a key building block for creating 3D assets of digital twins. With physically accurate simulations, we can represent reality in previously unseen ways, allowing prediction of various pathways of execution, behaviors and trajectories for better decision making, cost optimization and much more.
What is the role of AI in the metaverse?
Bednarz: AI is the future, and at NVIDIA we believe this deep learning will transform computing and other industries, boost efficiency in daily professional tasks, and improve lives in many ways. The industrial metaverse, the 3D evolution of the internet, requires a new programming model, a new computing architecture, and new standards — including AI support and industry-wide collaboration. The creation of the industrial metaverse will require developers, artists, designers, engineers and enterprises to work together.
The metaverse can be created either by hand or with the help of AI. In the future, it’s very likely that we’ll describe the metaverse using the characteristics of a house or a city. AI will create a new “city” for us — whether it’s like San Francisco, Toronto or New York — and if users don’t like it, they’ll be able to give the AI additional prompts or simply click a button to automatically generate another one. This “city” will be connected to the network of networks. Then, users will be able to modify that world. In fact, the AI for creating virtual worlds is already well under development. At the core is technology called large language models (LLMs), which can be trained on a broad range of knowledge and customized for specific use cases, including powering conversations and collaboration in 3D worlds. Soon, generative AI technologies will also help to create images, videos and 3D assets for these worlds. Creating advanced simulated worlds requires extensive amounts of training data. Since it can be costly, time consuming, and potentially not possible to acquire enough training data for a sophisticated AI system, simulation is frequently an ideal solution for securing this important data. As an example, NVIDIA builds platforms for self-driving cars, which need to be test driven for billions of miles and in situations that would be impractical to capture in the real world. Simulation is the only way to create all of the experiences needed for AI to train self-driving cars that can achieve full safety on the road. NVIDIA Omniverse enables users to aggregate physically accurate worlds, providing a framework to build simulators that are physically accurate.
What technology developments are fueling the advancements in AI, and how much of the human factor comes into play?
Bednarz: Advanced AI requires full-stack computing with integration and optimization from accelerated infrastructure to application-level software. Building advanced AI, including generative AI applications, requires accelerated computing and domain-specific software. Creating these applications from scratch requires significant resources and expertise, so NVIDIA offers a broad range of software and services to help enterprises customize foundation models using their own data to meet their unique business requirements. Synthetic data can be used to train models, especially in use cases like autonomous vehicles and digital twins, where real-world environments are simulated to train machines to operate safely across a broad range of variables once deployed in the real world. Ultimately, AI applications are built to serve the needs of people, with use cases that span healthcare, agriculture, climate research, communications and more.