Organizations in Asia Pacific are recognizing the need to unlock the value of the torrential amounts of data they generate.
Data scientists are undoubtedly one of the most in-demand professionals in the market. Data expertise is highly sought after, with organizations reimagining their business processes and seeking to harvest high-value insights from the vast amounts of data available today.
For instance, enterprises are increasingly turning to data to identify ways to eliminate inefficiencies, while using data visualization tools for reporting and planning purposes.
However, data scientists are often relegated to spending a significant portion of their time on tedious, mundane tasks such as data cleaning and preparation. There is little disagreement among data workers, including data scientists, that collecting, organizing, and cleaning data are the least enjoyable tasks they undertake.
What’s more, being a successful data scientist is not just about having the right technical skills but also about having a clear understanding of business requirements.
As more organizations acquire the ability to collect and store data inexpensively, they begin to see a need to build ML-powered data models. By moving their data onto the cloud, organizations can easily consolidate their data in a single location, enabling fast and secure data sharing and analysis to harness the full power of their data.
DigiconAsia approached Geoff Soon, Managing Director, South Asia, Snowflake,to share some tips for organizations and data scientists determined to succeed in this journey.
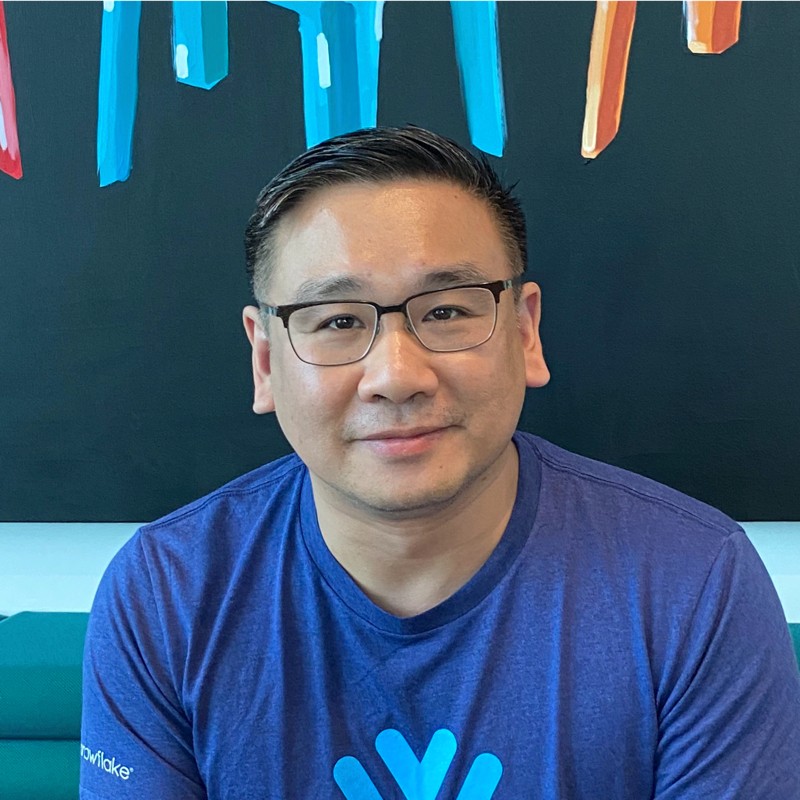
What are the top challenges data scientists face in their day-to-day work?
Soon: The number one challenge that many data scientists are facing is access to fresh meaningful data. A data scientist not only needs access to standard data but also understands how he/she is interacting with their organisation’s digital channels. For instance, a data scientist would want to understand how their marketing campaigns are working via data collected in their customer relationship management (CRM) system.
Due to the impact of COVID-19, the markets have changed more in the last 24 months than, arguably, in the last decade or so. Data scientists need access to third-party data to be able to refine their models. An example is how one of our partners utilises various COVID-19 data sets from the hospitals, the government, and the World Health Organization (WHO), and makes it available on Snowflake.
This is useful for data scientists because they would need to factor in things such as infection rates, death rates, and hospitalization rates related to COVID-19 to do any sort of advanced business planning. We have seen how South-east Asia businesses have been affected by COVID-19 cases almost daily.
Another challenge is how data scientists are able to access this data, which currently sits in silos, and adequately leverage third-party datasets when developing data science and machine learning models.
How can data scientists free their time and resources to tackle more complex data challenges and implement new technologies that move businesses forward?
Soon: An IDC-Alteryx study reports that up to 45% of time is wasted every week because data workers are unsuccessful in their activities. Given the challenges which data scientists face, some ways in which data science efficiency can be improved include:
- Taking advantage of machine learning frameworks:
It takes most data scientists about a month to perform feature engineering, manually run data against algorithms, and produce a new model. A more promising method is to devise reusable frameworks.
This method of working is increasingly supported by automated machine learning (AutoML) platforms. These platforms manage the data modelling process, removing much of the time-consuming manual work data scientists find themselves wrestling with every day.
- Operationalize AI using Machine Learning Ops (MLOps):
Frustratingly, many data science models don’t make it to the production environment. This is because there is often a disconnect between data scientists and their operations teams, causing misunderstandings and end results that don’t perform the way users expect.
A new practice dubbed MLOps is becoming more widely used to overcome this challenge, which involves operationalising the joint management of the ML data pipeline. When both teams focus on their respective strengths and work towards measurable outcomes together, data scientists are free to focus on business issues while ops teams manage the end deployment.
- Produce better insights by unlocking data:
When organizations use data lakes for long-term storage, they experience the challenge of unexamined and unused data. Although there is undoubtedly real value in huge data sets, the data must be unlocked so that it is easily discoverable, accessible, and usable. By moving data onto the cloud, organisations can easily consolidate their data in a single location, enabling fast and secure data sharing and analysis.
The best way to achieve this is to set up a self-service data refinery platform for internal use. Another method is to share data with outside organizations, such as partners, suppliers, customers, and vendors, and use aggregated data in a marketplace.
In short, data is rapidly transforming into an actionable asset. Data scientists and data analysts alike benefit from cloud technologies that provide virtually unlimited amounts of compute resources. When organisations manage their data effectively, they will then be able to harness the full power of their data to create and implement new innovative technologies that will strengthen their businesses.
What are the key skill sets needed for today’s data scientist (technical and soft skills)?
Soon: On top of having the technical skills to derive findings and insights, the most critical skill set needed is the ability to engage with the business function, as the fundamental role of a data scientist is to be able to sell a particular outcome to a particular business stakeholder.
Some soft skills that are critical in ensuring the success of a data scientist include:
- Communication
Communication is a key skill for data scientists who must be able to relay complex numbers and theories in a manner that’s easy to understand. Data scientists must also ensure that they can articulate their findings to business decision makers clearly and constructively.
- Adaptabilityz
Tech trends and innovations are constantly shifting. Data scientists must be comfortable with change so that they can adapt and respond to business needs accordingly.
- Critical thinking
Critical thinking enables data scientists to analyse data objectively. Data scientists are better equipped to understand and interpret data to solve problems and implement new solutions.
In addition to the soft skills, the technical skills laid out below are essential in strengthening the success of a data scientist:
- Knowledge of analytical tools
With a secure foundational knowledge of analytical tools, data scientists will be able to extract useful insights out of clean and organised data sets.
- Business needs analysis
Successful data scientists can effectively identify and analyse real-world business problems, proposing relevant solutions for the company.
- Data visualization
A familiarity with data visualisation software enables data scientists to create graphs or charts that are used to share their findings and help others better understand the data.
How can the data science talent gap in Asia be plugged? Can a public-private partnership enable a strong pool of such talent to bridge the current gaps?
Soon: The data science talent gap in Asia appears to be widening. Although 100 percent of Singapore companies surveyed in the WEF Future of Skills Report indicated that they were looking to accelerate the digitalisation of work processes, only 77 percent of the active population have the necessary digital skills.
In Singapore, there are public-private partnerships that address talent gap challenges. For example, the IMDA TechSkills Accelerator (TeSA) offers various programs that support professionals to upgrade their existing skills and acquire new ones. In doing so, they encourage professionals to stay competitive amid the challenges of a fast-moving digital landscape.
A key initiative in Malaysia is the MyDigital Alliance Leadership Council, which was designed to drive digital skill adoption in Malaysia. Leaders in academia, civil society, and the public and private sectors are collaborating to bolster the country’s competitive digital workforce, enhancing employability through transformative policies for education and skilling. That being said, the Malaysia Digital Economy Blueprint requires more private sector engagement to achieve its goal over the next five to ten years.
The demand for skilled scientists in tech will only increase as the region increasingly digitalizes. APAC organizations need to understand the importance of not only solving their skill shortages but also keeping their current skilled scientists satisfied. In addition, governments in South-east Asia have started to be more open in terms of the data that they share. As a result, private companies can leverage data such as the census and socio-economic data to enhance their businesses as well as develop capabilities to monetise it.