Unlike using general-use chatbots, building an LLM for searching internal data requires special finesse.
Few would deny that internet search has become an indispensable tool for daily life, helping us with everything from deciding on a recipe to choosing where to live. Everything is effectively searchable.
However, this ease of finding information does not extend to the office environment. All of us expend valuable time and energy trawling emails, spreadsheets, presentations and recordings to find what we need.
Increasingly, when it comes to internal knowledge or specialized areas specific to an enterprise, the AI may lack exposure to the relevant data, posing challenges in providing accurate responses.
Internal LLMs for business
For corporate use, the integration of LLM to build a relevant AI knowledge base presents two key challenges: application scenarios and data quality.
It is important to start with a clear picture of the usage scenarios and the problem to solve. We can think of it like a physician’s rehabilitation program, where the patient is the AI system and the program represents the carefully tailored application scenarios. Following this, we consider the data, which is akin to the medication in this analogy.
If the data is incomplete or unclean from the outset, the outcome from AI will inevitably be wide off the mark. The process of extracting data and reviewing the mode of operation, as in the case of a corporate health check, is the process of promoting digital transformation.
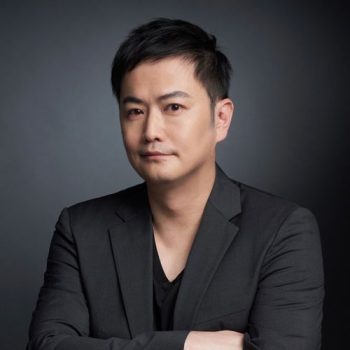
Kevin C.H. Lee, General Manager (Multimedia Technologies Business), KKCompany Technologies
However, merely forming digital assets is not enough. This is why comprehensive solutions for AI integration, ranging from asset management to knowledge management, and ultimately, to the application of its knowledge, are critical for today’s businesses.
In order to accelerate the introduction of AI and ensure contextual usage, enterprises need to analyze and reassemble various data types, and train these data types into large-scale language models that analyze each question and generate precise responses based on the content.
Two aspects to consider
With continual LLM and AI innovation, enterprises can combine video and audio streaming with generative AI to turn one-way viewing into interactive search experiences.
This will allow users to quickly find answers to questions and identify learning styles for self-directed learning.
In additional to proving this ongoing training to the AI, enterprises also need to consider two aspects around the LLM output information.
-
The first aspect is the personalized experience, which is becoming increasingly intuitive.
With AI, the recommendations will influence the way users interact with the content, whether through active questioning or passive suggestions. For users, asking questions through text input and receiving suggestions will be part of an ongoing relationship. Over time, the system will learn user-specific habits and preferences, so its recommendation service will become increasingly attentive.
-
Secondly, in the seamless transition of multimedia formats, information has to be presented to the user in the most appropriate manner.
This is because even rich sources of information can be fragmented enough to distract people’s attention, because it can come in multiple videos, social platform discussions and lengthy articles and studies.
Therefore, generative AI will need to be prompted to generate the LLM information that is styled according to the different needs of users on different platforms. This personalization will resonate with the target audiences effectively.
By applying LLMs and generative AI with the right approach, we can have the means to tackle the challenges associated with internal data search.