Predicting solar energetic particle events accurately and infallibly will boost progress in space exploration. The key is to ensure prediction explainability.
On 3 Feb 2025, Tokai National Higher Education and Research System, which operates Gifu University and Nagoya University, announced the commencement of joint research with the Japan Aerospace Exploration Agency (JAXA) on the “Development of explainable AI-based prediction model for solar energetic particle events for Moon and Martian exploration.”
The joint research had begun earlier on February 1, and will run until March 31, 2026. The aim is to use AI to work on data supplied by the Institute for Space-Earth Environmental Research (ISEE) and JAXA to improve prediction of solar energetic particle events (SEPs) on the lunar surface.
SEPs are a type of cosmic radiation that forms around solar flares and similar events. They propagate through space, and direct exposure to them on the lunar surface or in the outermost areas of space around the Earth can damage equipment and injure life. Accurate and timely predictions of SEPs can ensure the safety of all space activities, including lunar exploration.
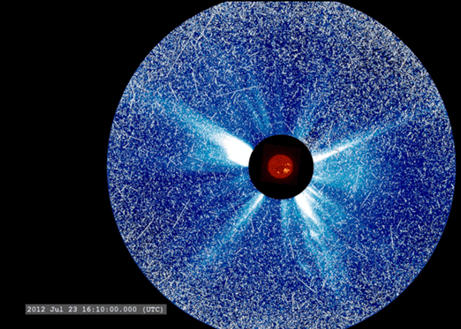
This type of advanced “space weather forecasting” is part of continual plans to explore the lunar surface, as well as JAXA’s Moon to Mars Innovation program focused on revolutionizing international space exploration. After 2025, space weather forecasting will be crucial to support a manned test flight and the first manned moon landing since America’s Apollo program.
Additionally, the space weather forecasting technology will be part of a “space radiation dosimeter” — a device scheduled to be installed on a manned lunar orbiting base that JAXA is developing.
A notable feature of the predictive AI technology used is the element of explainability: the AI platform supplied by Fujitsu is expected to explain all the causal relationships derived from the input data used for predictions. This allows for humans-in-the-loop management in tweaking and improving prediction algorithms, while also safeguarding against biases, hallucinations and other unpredictable AI errors.
The knowledge gained from the project is expected to benefit similar applications of explainable AI in the fields of space transportation and resource planning; emergency radiation warnings and even health management.