Can more public-private data sharing and AI/ML innovations be leveraged to end the cat-and-mouse game and boost financial inclusion and fairness?
As Southeast Asia continues on its path of rapid economic growth, the region is increasingly becoming a hub for financial crime, including money laundering, which poses a significant threat to the stability of financial institutions and systems.
In Singapore alone, despite the police investigating more than 19,000 money mules between 2020 and 2022, fewer than 250 cases were ever eventually prosecuted — due to various challenges in prevention, detection and digital evidence gathering.
One firm that is using AI and ML to combat financial crime and reduce inequality in the financial sector is Aboitiz Data Innovation (ADI), whose COO of Financial Services, Guy Sheppard, shares with DigiconAsia.net more information about some of the latest initiatives.
DigiconAsia: What is the secret sauce behind AI and data science that can detect mule activity accurately?
Guy Sheppard (GS): The key challenge around mule-account detection is that signals indicating a change in account use, legitimacy, and ownership can be very slight and often blended into the noise of daily monitoring; customer collusion is also rife.
Accounts that have been set up with legitimate documents and ID for genuine purposes are sold or hacked and misused, which bypasses existing Know Your Customer processes as the account is already “live”.
What we do to detect mule accounts uses a two-level approach:
- At onboarding, the AI model identifies the probability of a falsely set-up mule account and when the account is flagged, it further assesses account behavior and the likelihood of it being a mule.
- The AI-driven anomaly detection model continuously learns and improves to generate scored alerts of mule activity. This results in an improved accuracy rate in predicting risk of mule accounts.
DigiconAsia: What are the challenges that have prevented law enforcers from prosecuting financial criminals? How does technology help crime busters overcome those challenges?
GS: One challenge is the lack of private-to-private information sharing. The presence of bank-to-bank sharing clusters would help enforcers in acquiring the information necessary to detect fraud.
In Singapore, its central bank MAS launched an information-sharing program between six local banks to securely share information regarding customers that exhibit signs of potential financial crime concerns, making it easier to detect and deter financial crime.
- Additionally, most mid-size institutions still primarily use rules-based monitoring engines to identify “changes” in the behavioral patterns of account holders. However, these are blunt tools compared to AI/ML models that can more accurately distinguish true from false positives.
- Another challenge is that some financial sector professionals are not proficient in using AI to combat financial crime. They lack the technical skills to detect and deter such crimes, and their technology is not as sophisticated as that used by fraudsters. Therefore, education and training are needed to operationalize advanced tech into business operations.
DigiconAsia: How is AI/data science being used to help officials improve anti-gender-discrimination laws?
GS: A study based on non-mortgage fintech lending — conducted by ADI, the Union Bank of the Philippines and the Smith School of Business at Queen’s University in Canada, showed that using gender-related data resulted in a significant decrease in gender discrimination and an increase in profitability for firms. The data can serve as a guide to improve anti-discrimination laws to ensure that ML models foster a fairer system for discriminated groups within the financial services industry.
On the topic of AI bias: when using AI, it is crucial to have guidelines to ensure that the use is “explainable” (XAI) and responsible, revolving around main principles such as transparency, repeatability, human agency and oversight. This mandate also includes practical recommendations for those involved in the development of AI systems at ADI, and covers data collection and management, model development and testing, human oversight and control, and more.
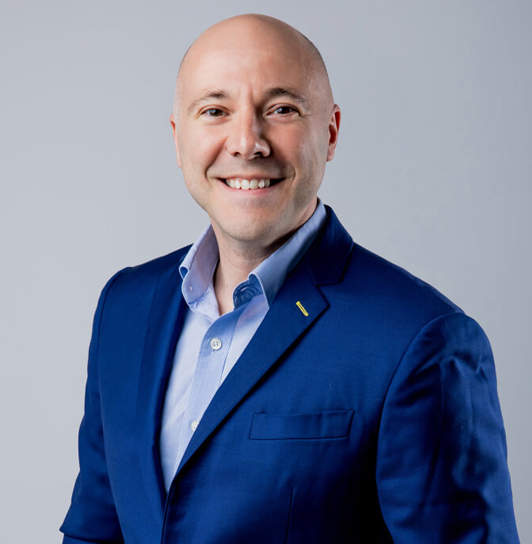
DigiconAsia: How are AI-powered alternative credit scoring models leading to increased financial inclusion among the unbanked and underbanked?
GS: Enhanced AI-powered credit scoring and risk models analyze various dimensions of customer behavior data, including minimal data submission and alternative data sources. This enables banks to provide loans and credit to individuals and small businesses who may not qualify under traditional scoring methods.
For example, in the Philippines, the use of alternative credit scoring and risk models can facilitate more efficient loan provision for the unbanked and underbanked population. In practice, by leveraging alternative data sets and AI-powered models, we have witnessed delinquency performance of bookings decrease by 11%, approval rates double, and booking amounts increase by seven times.
DigiconAsia: Cybercriminals now routinely fight AI and data science with the same AI and data science. Do you envision financial criminals eventually finding a way to foil AI detection or evade tracking? How about generative AI? Has this latest aspect of advanced AI democratized how financial criminals can escape detection, arrest and prosecution?
GS: The prevention of financial crime has become a game of “cat and mouse” in illicit activities in the digital space. Sophisticated criminal enterprises possess a deep understanding of banking environments, controls, and existing safeguards. They are also adept at designing software or misusing technology stacks to achieve their objectives.
Generative AI is a double-edged sword. While it can rapidly retrieve content from disparate KYC documentation and summarize the latest regulatory updates, it can also be used to crate deepfakes to bypass online account opening controls.
GenAI can also be used to reproduce an exact likeness of bank marketing and customer service messages to deceive users into downloading malware or sharing personally identifiable information and accounts.
This double-edged power has necessitated the use of “counter technology” to assign “liveness scores” for applicants applying via video link. The financial industry has made significant progress in sharing new typologies, allowing the collective to update and close the loop, especially through public-private partnership entities.
DigiconAsia thanks Guy for sharing his industry insights on explainable and responsible AI use.