Find out how the reliance on human-produced knowledge demands a robust strategy and FEAT to avoid pitfalls in AI adoption
Within enterprises, the integration of generative AI (GenAI) chatbots opens new avenues for employees and customers.
However, transitioning to this innovation is not simple, according to Lee Joon-Seong, Senior Managing Director, Center for Advanced AI Lead, Growth Markets, Accenture. Success hinges on data accuracy, completeness, and quality, echoing the principle of “garbage in, garbage out.”
In a Q&A with DigiconAsia.net, Lee expounded on how the reliance on human-produced knowledge demands a robust data strategy to avoid pitfalls.
DigiconAsia: Are the early adopters of GenAI and large language models facing any challenges in transitioning? If so, what solutions and prerequisites are needed in place to facilitate a smooth transition?
Joon-Seong (JS): Businesses can consider the following principles to integrate GenAI and large language models (LLMs) seamlessly throughout their value chain.
- Lead with value: Shift the focus from siloed use cases to prioritizing business capabilities across the entire value chain based on the return on investment. Organizations need to be value-led in every business capability that they choose to reinvent with GenAI. It is recommended to pursue investments in two categories: firstly, “table stakes” investments that offer radical efficiency; and secondly, strategic bets that stand to bring truly novel advantages.
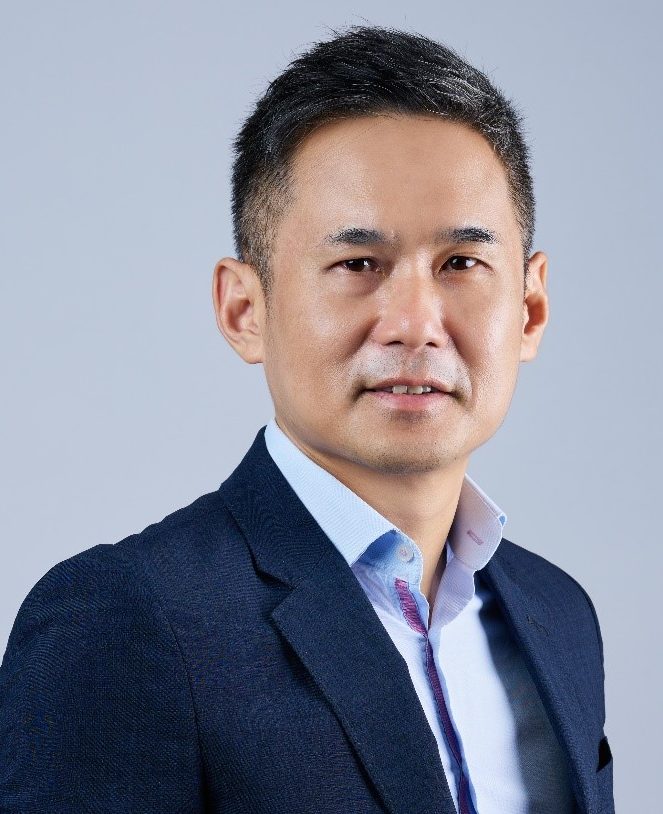
- Understand and develop an AI-enabled secure digital core: Enabling a modern data platform is very important for success. Re-architecting applications to be AI-ready is also critical, with a flexible architecture that allows you to access a range of models in partnership with the ecosystem. This security is a foundational element for protecting personal or proprietary data.
- Reinvent talent and ways of working: Adopt people-centric approaches to GenAI adoption and innovation. To prepare workers for further integration of AI, organizations must adapt existing operating models for new ways of working. For example, adopt new techniques and high-performance equipment; investing in modernizing technology for a secure digital core; emphasizing people in all reinvention strategies, such as new leadership training, involving people in design, and upskilling them on GenAI.
- Close the gap on responsible AI: Another imperative is to design, deploy and use AI to drive value while mitigating risks. This includes the strategy and development of responsible AI monitoring and compliance, as well as employing AI security into the entire value chain. For example, ensure all stakeholders can assess their AI and data analytics solutions based on fairness, ethics, accountability, and transparency (FEAT) principles. Demystify GenAI complexities and draft a risk framework for its responsible integration.
- Drive continuous reinvention: Given that GenAI-led reinvention journeys are complex and multi-year in nature, firms need to be prepared to allocate capital, time and talent for the long term. To do that, embrace a modular, step-by-step approach to innovation that spans multiple years. In addition, cultivate a corporate culture that views continuous reinvention not just as a strategy but as a core competency.
DigiconAsia: What are the universally accepted principles for creating an accessible and contextual data foundation on which to base LLMs on?
JS: Organizations need to enhance IT capabilities for the AI era with a strong and secure digital core. This will require a modern data foundation and flexible AI architecture, allowing the use of multiple foundation models while future proofing against potential model changes.
Furthermore, they also need to consider whether they have the right technical infrastructure, architecture, operating model and governance structure to meet the high compute demands of LLMs and GenAI, while still keeping a close eye on cost and sustainable energy consumption.
Therefore, it is imperative to assess the cost and benefit of using these technologies versus other AI or analytical approaches that might be better suited to particular use cases.
Next, customizing foundation models will require access to domain-specific organizational data, semantics, knowledge, and methodologies. In our research, respondents succeeding in AI were 2.4 times more likely to store data in a specialized modern data platform in the cloud.
As foundation models require extensive curated data to learn effectively, a strategic and disciplined approach is necessary to acquire, grow, refine, safeguard, and deploy data. Specifically, organizations need a modern enterprise data platform built on cloud with a trusted, reusable set of data products. Due to cross-functionality, these platforms facilitate breaking data free from organizational silos, democratizing AI use through enterprise-grade analytics, and housing data in cloud-based warehouses or data lakes.
In terms of sustainability, AI usage could increase the carbon emissions produced by the underlying infrastructure. Organizations need to invest in sustainable tech foundations, such as a robust green software development framework that considers energy efficiency and material emissions throughout the software development lifecycle.
DigiconAsia: How do organizations address any new or existing risks in adopting GenAI?
JS: There are a few key GenAI risks organizations need to consider, such as misinformation, inaccuracy, the security of proprietary and confidential information and even workforce displacement and readiness. Organizations need to be more intentional in protecting themselves from these risks, and taking on a responsible AI approach is ideal.
For example, to counter misinformation, executives should define and articulate a Responsible AI mission and its principles. This includes establishing a transparent governance structure across the organization that builds confidence and trust in AI technologies.
Our own research indicates that: while most CxOs we surveyed believe in the benefits of GenAI, only about a quarter actually had comprehensive strategies in place to ensure positive worker outcomes and experiences. In fact, about half of affected workers were concerned about job displacement. This underscores the importance for organizations to empower their leadership to prioritize Responsible AI as a critical business imperative. Mandating training for all employees ensures a clear understanding of Responsible AI principles and criteria for success.
Regarding unethical AI use, the risk of malicious use of AI technologies will always be present. We are already seeing this with applications such as FraudGPT, WormGPT and DarkLLM. What is crucial here is raising awareness, staying informed and vigilant.
Leaders and employees need to be highly attuned to the business and security risks that their organization may be incurring, and more importantly, aware about how those risks can be minimized. There are ever-evolving models, frameworks, and technologies available to help guide AI programs forward with trust, security and privacy throughout. Focusing on trustworthy AI strategies, trust by design, trusted AI collaboration and continuous monitoring help build and operate successful systems.
Transparency and trust are crucial for people to effectively adopt and embrace these tools — organizations will need to integrate AI in ways that protect and prepare workers. Organizations need to develop a framework to unlock people’s full potential by meeting fundamental human needs, to close the trust gap and get people ready for AI. Ultimately, we believe that AI will not replace humans, but humans with AI will replace humans without AI.
DigiconAsia thanks Joon-Seong for sharing his insights with readers.