Having more open, sustainable cloud infrastructures that lower barriers to entry, in terms of cost, accessibility and flexibility, can democratize GenAI
AI is becoming a de facto tool for everything from research and development through to production, post-sales services and achieving sustainability goals.
We are already seeing this with generative AI (GenAI): Large language models (LLMs) have clearly captured the imagination of organizations, and they are now accelerating interest in further GenAI capabilities internally and also through third party applications.
However, what if an organization cannot connect all of its data or cannot scale its infrastructure? What impact does that have on its ability to take advantage of GenAI?
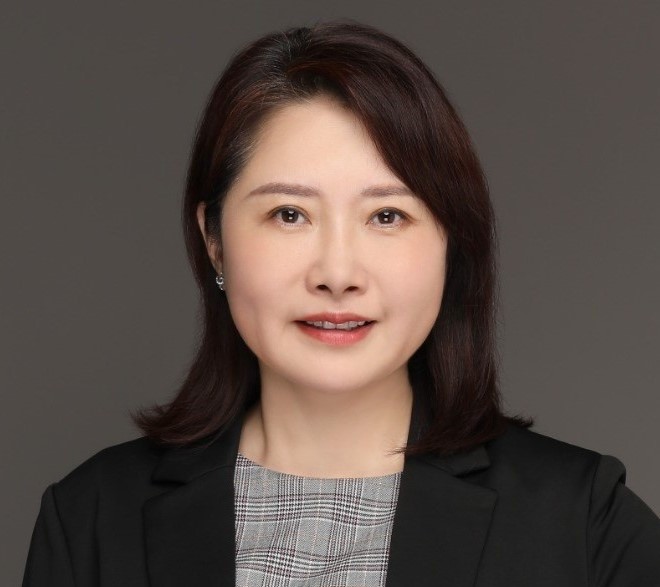
The Cloud-to-AI cost factor
Cloud computing, of course, becomes crucial here. How many businesses are actually being held back by budget constraints or the complexity of managing and updating disparate systems? Not all cloud infrastructure is equal. There needs to be a leveling of the playing field to give as many organizations as possible equitable access to empowering technologies.
Basically, for organizations to truly reap the benefits of GenAI, there is an urgent need for a more open, sustainable cloud that lowers barriers to entry, both in terms of cost but also in terms of flexibility and access. The world needs cloud pricing strategies that are designed to benefit long-term subscribers with significant discounts while also providing businesses with a stable foundation for developing their long-term strategies in planning and creating their AI applications.
Through affordable access to GenAI capabilities in the cloud, more organizations will be able to improve returns on investments in AI technology.
Democratization for the GenAI boom
Over the next few years, cloud infrastructures need to be designed to be GenAI-centric, to drive innovation and action — with clear cost structures and scalability. Through an open cloud infrastructure, businesses can be empowered by greater transparency throughout the model-training process, as well as improved interpretability of AI algorithms. How?
With democratized AI and cloud infrastructure, organizations can then address data issues without worrying about the underlying infrastructure. There should not be trade-offs. Cloud infrastructure has to become a de facto standard, forming a baseline for organizations to operate LLMs, to experiment, innovate and grow.