In India, the rich can borrow money to get ‘richer’; but technology may soon give hope to poor but credit-worthy people…
Recently, Maharastra state police arrested three persons allegedly running a fake-loans racket in Pune, Thane and Navi Mumbai targeting people with a low Credit Information Bureau of India Limited (CIBIL) score.
The police said that the accused persons charged ₹5 lakh in cash as commission for sanctioning loans of ₹1 crore to ₹100 which never materialized after payment.
The scam exploits the divide that CIBIL scores creates among people, singling out those who are deemed by the financial system to be not trustworthy to be issued loans. And the number of such scams is rising in numbers.
Can the CIBIL divide be addressed with AI and analytics? Nageen Kommu, founder and CEO, Digitap, shares his insights with the DigiconAsia.net team
DigiconAsia: Since the CIBIL score points to the financial discipline of an individual or an organization, can better AI and analytics mitigate the perceived risk they pose when they require official loans?
Nageen Kommu (Nageen): If one depends on the credit bureau score completely, then all financial institutions will be targeting people with a higher-than-730 score.
However, among a large portion of Indians who have a low bureau score and will not have access to credit, other positive circumstances may point to them having a good propensity to repay loans under certain circumstances.
The use of AI, when applied in an inclusive manner, can help financial services firms to identify this group of people. This would involve the use of a risk management stack fed with extensive ‘alternate data points’ that can offer a more granular approach to appraising credit worthiness. Additionally, any residual risk undertaken can be automatically monitored and managed in the customer onboarding and subsequent loan period to affirm or disprove any trust accorded to people who would otherwise never have been offered any loans.
DigiconAsia: What are the key alternate data points and their role in helping an AI-driven credit decisioning system to manage risk assessment and monitoring?
Nageen: We have identified the following key data points for a customer that can be used for effective underwriting:
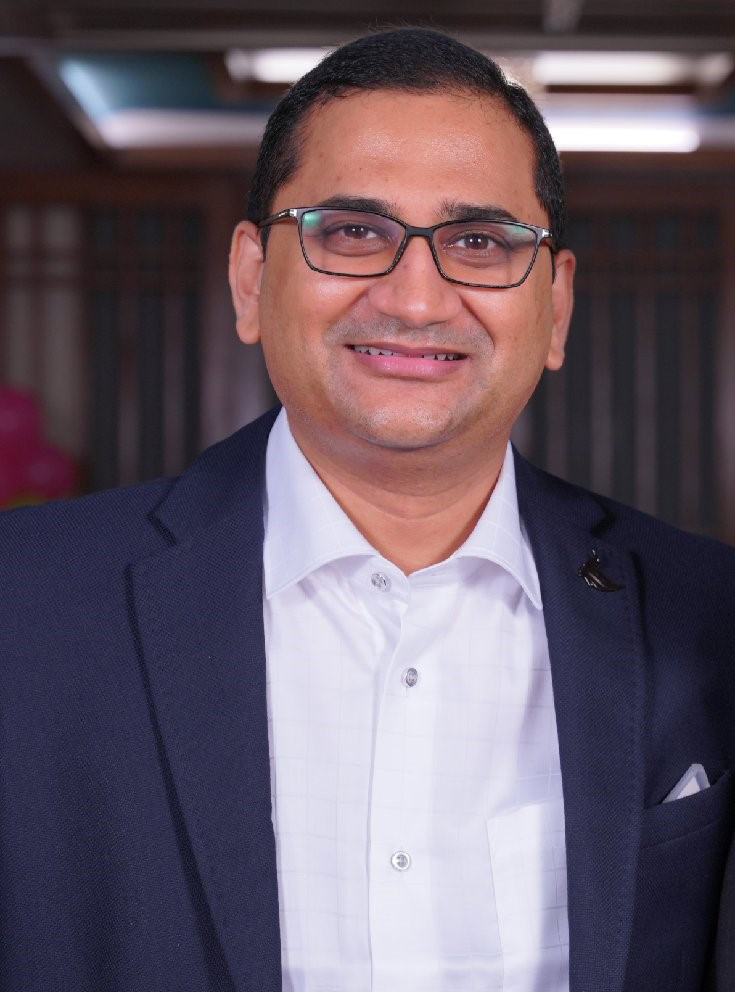
- Bank statements
- Device data
- Ecommerce data
- Employment data
- Location
- Telecom data
- Social network data
Some of these data points are useful to identify whether to grant credit or not; others are useful to answer the question of “how much credit to grant”.
Based on the past and future behavior of any borrower across these various data points, an AI credit decisioning system will be able to identify accurately (and hopefully vindicate) the credit worthiness of people who have now been allowed to be customers.
DigiconAsia: Tell us more about the trend of using technology to boost financial inclusiveness in a country of 1.4bn people.
Nageen: India is striving towards financial inclusion which means enabling access to financial instruments for all its citizens. For such a huge diverse population, this can only be possible if the
the banking, financial services and insurance (BFSI) sector uses AI and analytics to automate credit decisioning.
A data driven approach enables the automation to run efficiently and effectively, thereby enabling the financial institutions to serve the huge population with ease. In terms of scalability and efficiency, this approach helps the BFSI sector to make accurate decisions at scale, reduce processing times and improve continual risk management.
DigiconAsia: What challenges stand in the way of this making this data-driven approach work?
Nageen: In terms of risk assessment, the major difference between India and the rest of the democratic world is the availability (or lack thereof) of data for underwriting purposes.
In the rest of the world, the data is readily available in a structured format for the financial institutions to analyze it and make decisions with. In India, the availability of data in itself is a challenge. Next, even if the data is made available, putting it in a structured format for running analytics could be a huge hurdle.
DigiconAsia: How accurate have AI-driven credit risk assessment been in India so far?
Nageen: Accuracy levels for the AI-developed scoring models have depended on various factors such as data availability, data structure, and the analytics engine that is run on top of the big data.
India lags behind in the area of data availability, making it difficult to achieve 100% accuracy levels in assessing credit risk. However, the government has also taken steps to standardize the data structure and make data available through secure means, via the Account Aggregator framework, which is described well by the Indian Express.
We believe that the overall accuracy levels will dramatically increase once the framework is well established in the country. Going forward, data analytics and AI will play a crucial role in India’s BFSI segment when we move away from product centric approach to the customer centric approach.
Financial institutions will need accurate data (including alternative data points) to be available, to not only make credible decisions about customers and potential customers, but also on the quantity and type of products that can serve customers of various risk profiles well.
DigiconAsia thanks Nageen for sharing his insights on India’s credit landscape.