Reliance on a limited pool of data science specialists delays decision making, which is detrimental in an environment where speed and agility are key to business success.
Over the past year, COVID-19 has accelerated the use of technology, and the demand for data skills has grown exponentially.
A LinkedIn study found that data science was one of the top skills on the rise, with data science specialist roles experiencing one of the highest year-on-year growth rates between April and October 2020 in the region’s business hub Singapore.
The demand for data skills is not surprising. As organizations collect increasingly diverse sets of data, the analytics use cases to transform data into valuable insights are growing just as quickly.
The problem is that not every company has access to a specialized data science team, and those who do often have a small, highly skilled team with a huge backlog of projects. More often than not, we see business users and analysts with domain knowledge and proximity to business data, but they lack the tools or technical skills to do advanced, statistical analysis or to manage machine learning projects themselves.
This reliance on data science specialists delays decision making, which is detrimental in a business environment where speed and agility are key to business success.
DigiconAsia sought out some insights into the challenges and possible solutions from Andrew Beers, CTO, Tableau:
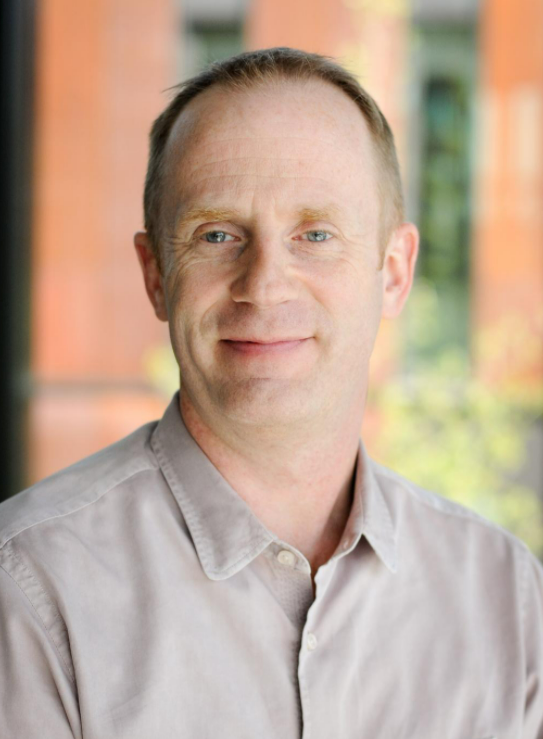
Is there an increasing demand for data skills in Asia Pacific, and are we experiencing a major skills gap in this field?
Beers: Data skills are definitely in high demand — not only in Asia Pacific but worldwide. And we’ve seen a sharp increase in that over this past year as organizations shift to more digital experiences. In Asia Pacific, McKinsey reported the adoption of digital services accelerated by 10 years due to COVID-19.
All this change is creating a huge influx of new data— data representing the essence of an organization including customer needs, finances, and managing employees. This new data presents immense potential for organizations if they can unlock its value and put it to work.
Still, organizations are struggling with the infinite amounts of data coming in. There’s simply not enough people who understand how to harness all this data to drive transformation. In fact, a report by AWS earlier this year revealed that up to 819 million workers in Asia Pacific will need to possess digital skills in data, cloud and cybersecurity to keep up with emerging technologies by 2025, up from 149 million today.
It’s becoming increasingly clear that we have a data literacy problem. The ability to read, understand, and use data will be a critical skill for everyone in this digital-first world going forward. Acknowledging this educational gap and addressing it fast must become a civic priority, not just an academic one. Technologies like AI and Machine Learning will also help make data and analytics easier for everyone to use, but it’s just part of the equation. Organizations must also invest in data and analytics so that it becomes a core competency for everyone.
What is Business Science, and why is there a growing need or demand for it? What value does Business Science bring to businesses (with examples for specific industries)?
Beers: To be transformative, organizations need agility and that comes from making the right decisions fast. But there’s simply too much data for many organizations to handle and not enough data people. This dilemma has sparked new trends — one of which centers on data science and it’s becoming imperative to business transformation.
Data science is a critical part of the analytics process, and data science teams are great at producing precise models, which are often then used to drive automated processes that accelerate transformation. But oftentimes these data scientists are maxed out working on issues with the highest priority, yet people all across the business still need help to solve contextual problems that require some data science but don’t justify–or can’t wait for–a data scientist.
Tableau Business Science uses technologies like AI and Machine Learning to expand data science capabilities to more people so they can make better decisions faster — regardless of their technical expertise. It gives people with the right domain expertise and business context the ability to build predictive models, plan simulations and scenarios, and cluster data.
This means more people across the business are better equipped to tackle tough questions like resource allocation, prioritization, staffing, and logistics. For example, taking a data-led approach to marketing and sales with opportunity scoring, predicting time to close, and many other CRM-related use cases that most data science teams can’t prioritize are highly valuable.
Or a sales team might use predictive modeling to determine the most profitable upsell opportunities. An algorithm can offer predictions about the likelihood that a customer will buy, though it doesn’t have the critical knowledge of an account executive managing the business relationship. But if the account executive can tap data science capabilities using AI, then they can explore various possibilities and see which scenarios will best help their customers achieve their goals.
This also frees up time for data science teams and allows them to tackle the really big, mission-critical problems. Traditional data science processes might be overkill for most business questions since deploying and integrating traditional custom models is complex, requiring statisticians and data scientists to make solutions consumable and actionable by end-users. Business Science empowers people to create and iterate on predictive models which can enhance their ability to think fast and act with confidence.
How important is the role of AI and ML in efficient decision-making, and how can we make AI-based decision-making more ethical?
Beers: Perhaps the real magic behind Business Science is how it proves that AI can enhance people’s unique perspective and experience to drive better decisions faster. Simply put, AI can help people do more with data.
And over this past year, we’ve even seen organizations across every industry use data to make powerful transformations happen. All of this work is brilliant and there’s still so much more we can do with AI. It can help reduce bias in decisions, and ensure data is used responsibly and ethically.
But we wouldn’t want AI to make all our decisions for us. People bring a wealth of diverse perspectives, creative thinking, contextual experiences, and ethical judgement to decision-making. And the ability to think critically and ethically about data – having the data skills to recognize biases or errors in the analysis – is more important than ever.
Data management is another key piece to help ensure data and technology are used responsibly and ethically when making decisions. Because if you want to build lasting trust in the data and its usage, you’ll need to manage all of this data with the appropriate security and governance required to responsibly deploy and scale AI-driven analytics across your organization.
Where does Business Science fit in the evolution of Smart Cities?
Beers: Tableau has had a longstanding commitment to the public sector in Asia Pacific. In fact, we work closely with GovTech, a lead agency driving Singapore’s Smart Nation initiative which helped make Singapore one of the smartest nations in Asia.
Through our partnership with GovTech, we’ve seen the transformative power that data science can bring to the public sector. And with Business Science, government organizations and agencies can expand their data science capabilities beyond data science teams to public officers and policymakers. At the same time, data scientists in government can focus their efforts on uncovering deeper insights — accelerating the transformation of public services and operations in urban areas.
For example, population growth and immigration can put a strain on existing resources available. Urban planners need to paint a forecast of the future when recommending policies, programmes, and new technologies to adopt. By tapping on historical data, data science teams can use AI and ML technologies to build predictive models of what the future city can potentially look like. Such insights can then inform decisions relating to risks and costs in Smart City projects.
However, relying on historical data alone is not enough to determine how our fast-changing environment might affect these projects. Input from domain experts is also necessary.
This is where Business Science comes in. It enhances collaboration between data science teams and other stakeholders involved in the city planning process. Data scientists can continue to provide custom models and statistical analysis but partner with other stakeholders such as policymakers to validate the data being used in ML-powered models.
Business Science also empowers other experts such as policymakers and architects to leverage the predictions and insights that come out of ML models and do analysis themselves to finetune urban plans along the way. They can lean in on their human judgement and knowledge in related domain areas such as building and construction regulations and emerging design trends to determine how such factors can affect the city landscape in the future.
We believe that Business Science will be a critical element to the evolution of Smart Cities around the world.