Looking ahead into 2021 and beyond, the next stage of automation evolution could lead to a marked shift in how workers view automation.
Over the next five years or so, we will start to see a marked change in the way automation impacts our working lives and how it is perceived by workers. As AI and robotics evolve, automation will become more accessible to more employees, easier to use and democratized in a way few can imagine.
Five years ago, few of us could believe that with a few taps of our phone, a car will pull up to take you somewhere, or someone will bring you dinner. The next major change will happen in workplace.
While experts from both sides have been either decrying how AI and automation are displacing people from jobs or assuring employees that the technologies would help increase their productivity as well as create new jobs, what would put our hearts and minds to rest on the issue?
For some answers and a peek into what automation in the future workplace looks like, DigiconAsia sought the views of Chris Loo, Managing Director, Southeast Asia, UiPath:
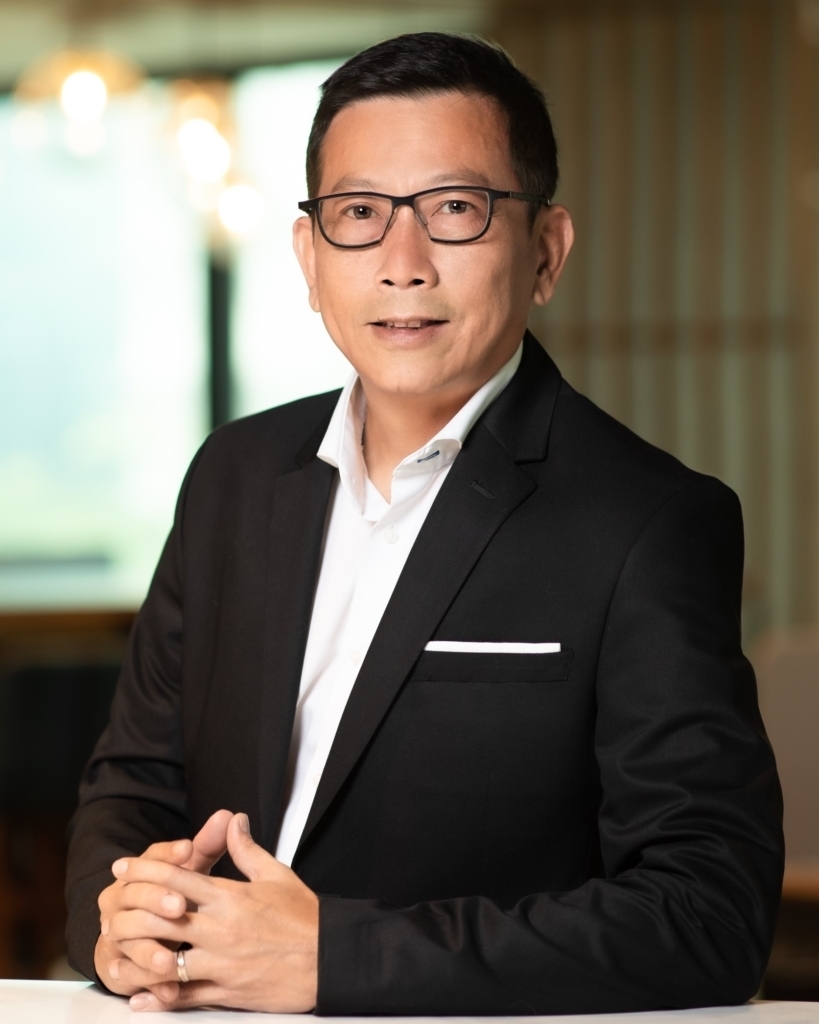
What, in your opinion, is the next big step in automation at the workplace?
Loo: I see the next big step to be the end-user – in other words the employee – to be able to easily and quickly automate tasks, aided by machine learning and Artificial Intelligence (AI). Traditionally, Robotic Process Automation (RPA) has been the preserve of IT departments and senior leadership who have leveraged the technology to generate more efficiencies, transition employees into more value-added work and become more adaptive and flexible on a company-wide level – it was always a ‘top-down’ approach.
This has worked very well to date, but as the technology further evolves, we will see a much more bottom-up approach whereby the workers themselves will be drivers of automation.
I foresee apps that can be downloaded, much like you would your Uber app, that will allow employees to automate daily tasks and connect end-user applications to enterprise-wide applications. Process and task mining will collect user data, analyze it, and then the robot will be able to say ‘Hey, I notice you do this activity repeatedly on a daily basis, I can automate this’, much like a Siri for automation.
The result will be employees who are empowered to improve their own productivity and automate tasks that are suited to them. This in turn will permeate throughout the company, with innovative and interesting ideas emerging from deep within the company that can be scaled throughout the enterprise.
How would advances in AI impact the way we work with machines today and in the near future?
Loo: AI, working alongside task and process mining, will play a key role in guiding what we automate. I mentioned earlier the ‘Siri’ for automation, whereby your app will be able to proactively advise what you can automate in your daily life based on data. This will be powered by AI and is a huge step forward from the back-office work that AI is currently being used, alongside RPA.
I foresee a robot assistant for every employee in the near future, where they will work as a team to get jobs done. Importantly, this will lead to better quality jobs. One of the challenges many companies face today is that employees simply do not want to do the repetitive, rules-based work, they want to add value, use creativity and innovate. RPA, combine with AI, will allow this to happen.
When conceptualizing RPA and AI, it can be helpful to think of AI as the brain, and RPA as the hands. It’s when the two are combined that more complex processes are automated. AI brings RPA to a new level. When you first learn about RPA, you’re told to look for processes that are rule-based, uses structured input and doesn’t require human judgment. With AI, processes that have some ambiguity and deals with unstructured or semi-structured input can also be automated.
With advances in AI, more companies are able to expand their omni-channel strategy to include chatbots in their effort to reduce costs associated with running their contact centre. We already see real-life examples of how we interact with chatbots with day-to-day transactions that we perform with telco and logistics companies. There are telcos that are now allowing you to re-contract your mobile plan or switch to a different plan through a chatbot. Furthermore, logistics companies are also allowing you to track your deliveries or reschedule deliveries through chatbots.
In the digital economy, where speed and security are both critical when it comes to application development, is low-code the way to go? What are the benefits and potential pitfalls?
Loo: Low-code application development is all the rage as more companies, large and small, rush to digitalise their business. One of the things that make low-code development appealing is the IT department can let users create their own app. The ideal situation is that you have the IT department choosing a secure platform for low-code development and setting a governance framework for how users develop applications.
But with the relative ease at which lines of business can get access to low-code platforms without IT involvement, the emergence of shadow IT could expose companies to security risks. It is not uncommon to see large companies leverage different low-code platforms which results in the proliferation of undocumented applications.
Companies should create a Center of Excellence (CoE) team staffed with representatives from LOBs and IT. Some of the responsibilities of the CoE should be to create standardisation, set up the governance framework, promote reuse and collaboration, and raise security awareness of all stakeholders.
How should low-code application development platforms and AI work together for our benefit – both as a business and as a consumer?
Loo: The main challenge with AI that most enterprises face is around operationalizing and monetizing their AI capabilities.
When the Data Science team has completed the development of a model, operationalizing and monetizing the model will require the application development teams to make changes to existing applications or build new application that leverage the capabilities of the model. But with traditional application development approaches, this could take months or sometimes years to come to fruition. Low-code application development and RPA can operationalize AI in a much more rapid manner.
Let’s take the example of a contact center. Let’s say we aim to reduce the average handling time of calls and improve the ability of our agents to upsell new products. We commissioned the Data Science team to create an AI model to predict what product or service would interest a particular customer given his profile and transaction history. This is usually referred to as the “next best action”.
The traditional application development approach would call for the modification of our existing core applications and CRM system to incorporate the AI model. However, with low-code application development and RPA, we can build a unified front-end that can be used by contact centre agents so they can see all the information about a customer on a single pane of glass.
Robots assist in the background, extracting customer data from applications by making API calls, database calls or by scraping data from front-end of applications. When a call from a customer is received, the AI model is triggered automatically by RPA to provide the next best activity or recommended action. The prediction given by the model would then be displayed on the unified front-end so it can be offered by the contact center agent.