Amid the surge in AI investments across Asia Pacific, many organizations could be overlooking a critical element that could determine their AI success or failure.
While the spotlight on AI for many organizations in the last one to two years has been on generative AI tools and training LLMs, data is the real game-changer, according to Qlik.
DigiconAsia discussed the real-world practicalities and examples of data management in an increasingly AI-driven world with Andrew Clarke, General Manager and EVP, Data Business Unit, Qlik.
“Effective AI depends on lots of data, and quality AI relies on quality data.” To what extent is this true, in your opinion? What should be the key data-related considerations for organizations gearing up for AI?
Clarke: I cannot agree more with this statement. Fundamentally, good AI is built on relevant and quality data. Without careful attention to the data we use, AI can return results that are incomplete, false, or biased. This can create major ethical and reputational risks for organizations and limit their real potential with AI.
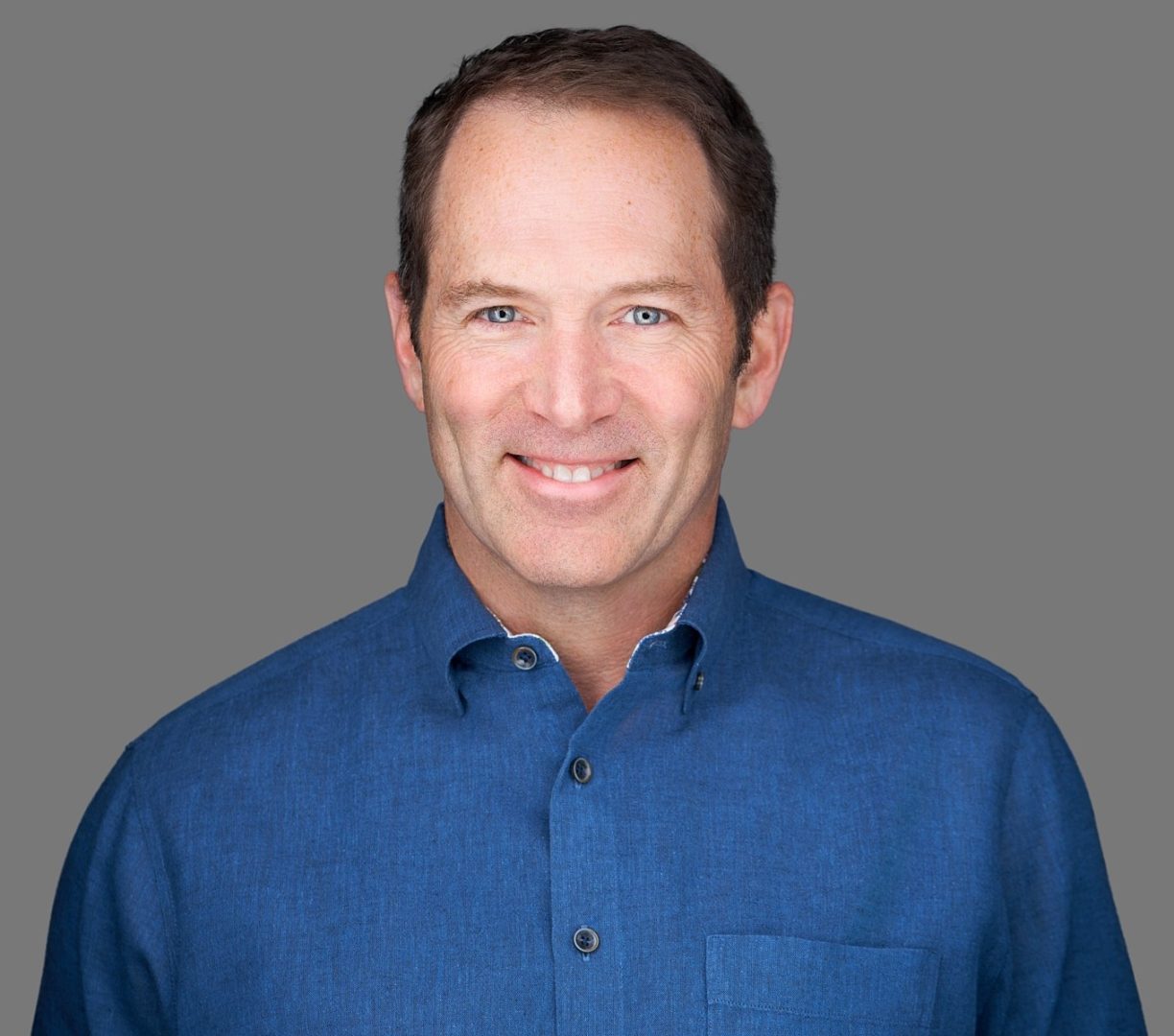
To create truly effective and quality AI outputs, it is on organizations to get their data house in order. An important consideration is committing to a process of accessing high-quality, well-harmonized, and accurate data supported by a scalable data architecture, with proper governance and security measures in place.
For example, with data integration capabilities and utilizing Qlik’s Singapore cloud region to store and deploy data for analytics locally at scale, Singlife’s claims process has been streamlined, leading to a three-fold increase in efficiency and a 35% reduction in data analysis costs. With the landscape of data management set to become even more integrated with AI, scalable and well governed integration is how organizations can unlock value from their data.
As a first step, organizations should prioritize data quality through stringent integration and governance practices. Data is typically moved from the source of origin (like applications, transactional databases or IoT systems) into a variety of data management systems such as a data warehouse, data lake or data lakehouse. Using a federated approach, data no longer must be centralized to be utilized. Fit for purpose data products can now be defined for specific organizational domains regardless of where the data resides which accelerates time to value, reduces cost and promotes greater reuse.
Synthesizing data from different sources will strengthen the overall quality and robustness of AI algorithms by reducing biases and other limitations that may arise from relying on a single dataset. With quality data, organizations can create effective AI solutions to accurately identify trends, make predictions, and improve overall operational efficiency.
How can organizations in Asia Pacific ensure they have a trusted data infrastructure for their forays into AI?
Clarke: Data is growing exponentially in the era of AI. If organizations can manage and utilize their complex datasets efficiently, they will be able to make strides towards enhancing the trustworthiness and reliability of their data.
In fact, 70% of workers in a recent McKinsey survey said they have experienced difficulties integrating data into AI models, citing challenges with data quality, processes for data governance, and having sufficient training data. If left unaddressed, this can lead to severe consequences, including operational failures, regulatory breaches, and financial losses. Robust data governance, high-quality data, and effective data integration are key ingredients for a trusted data infrastructure.
To overcome these challenges, organizations must establish comprehensive guidelines for data management, embrace advanced data quality tools, and use metrics to continually assess and improve data quality.
Airbus is a prime example of how improved data quality can enhance an organization’s productivity. By consolidating over 15 data sources and ensuring various business users like design teams and production teams can access the data in one place, Airbus accelerated its aircraft production to meet growing demand.
Tools like Qlik Talend Trust Score are also accessible for organizations to help monitor the quality of their data. By implementing these practices, they can build a reliable and effective data infrastructure that supports successful AI initiatives.
Are the regulatory regimes in various countries hampering or helping the adoption of AI in businesses?
Clarke: Regulatory regimes can significantly influence AI adoption in businesses. The impact varies depending on the country and its approach to AI regulation. In countries like Singapore, there are clear and useful guidelines like the Model AI Governance Framework to provide businesses a structured approach to adopting AI compliant with local data regulation and ethical standards. This accelerates AI adoption in the country by providing a safe space for innovation and encouraging experimentation.
Not all countries in the region have such readily available guidelines. According to IDC, only 22% of large Asia Pacific government agencies have implemented comprehensive, responsible AI governance policies. This is where industry leaders will play a pivotal role in shaping the future of AI governance, bridging the gap between technological innovation and regulatory standards.
The recent Qlik Generative AI Benchmark Report, notes the sheer scale of investment in AI: 31% of senior executives plan to spend over $10 million on generative AI initiatives in the coming year, and a significant 79% have already committed resources to AI tools or projects. This level of enthusiasm signals a strong belief in AI’s transformative potential.
But enthusiasm must be paired with effective data management. The report makes it clear: the success of AI depends on solid data strategies. Without proper governance, the efficiency and integrity of our operations are at risk as AI becomes more prevalent.
To tackle these challenges, we’ve formed the AI Council to guide Qlik’s R&D, shape the product roadmap, and ensure that AI initiatives adhere to ethical standards. We’re focused on educating Qlik’s leaders and employees on leveraging AI effectively while meeting business leaders’ needs to demonstrate the value of their AI investments. This initiative will help us navigate AI complexities with strategic insight and ethical commitment.