Many organizations are still navigating the challenges and frustrations of GenAI implementation. What’s gone wrong?
Over the past year, there has been a growing frustration among business leaders surrounding the integration and utilization of this GenAI technology. Businesses across various industries are grappling with the complexities of AI implementation and one of the primary sources of frustration is the constraint posed by legacy data infrastructure.
Many organizations have invested heavily into GenAI, yet they struggle to achieve meaningful outcomes due to the limitations of legacy infrastructure, with only 35% having moved beyond initial stages with ready data access – leaving the remaining 65% of stakeholders and end users without ready access to usable data.
DigiconAsia sought out some insights into how organizations can overcome these fundamental challenges of GenAI implementation and how a shift away from traditional data architecture is key to maximizing GenAI efficiency in this Q&A with Robin Fong, Regional VP & GM, ASEAN & Korea, Denodo.
The emergence of GenAI has changed the way organizations harness and leverage data. However, there seems to be a growing frustration among business leaders surrounding AI implementation. What could be the causes of this frustration?
Fong: Ever since ChatGPT was launched in late 2022, AI has taken over the business world. Having seen early success with basic use cases (e.g. chatbots and summarization functions), businesses are hyped about GenAI. That is an undeniable fact. But just because organizations are keen to implement AI, does not mean that their data is.
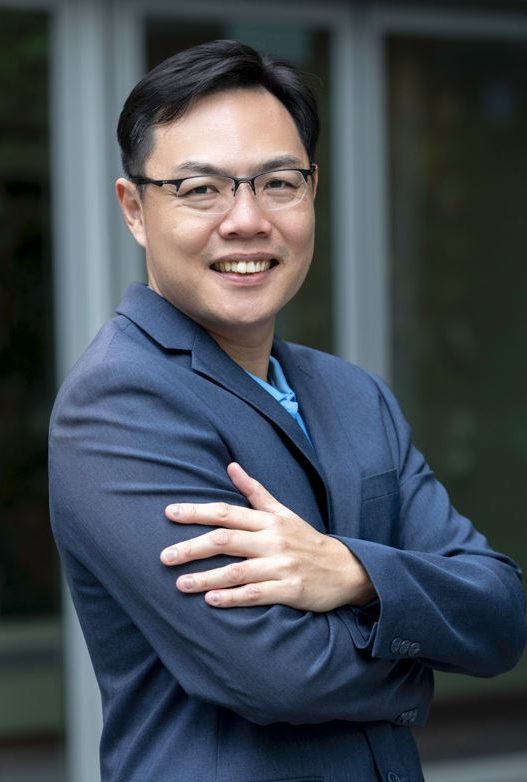
Companies are quickly realizing that their current data foundations are ill-equipped to cope with the vast volumes of data processing required by Gen AI solutions to make them actually impactful, while still adhering to data security and governance. Too often, businesses keep their data in silos, in multiple different data pools, and this results in a lack of ready data access. Then, when these companies try to integrate GenAI use cases across their business, they find that the use cases have limited impact and accuracy due to the GenAI’s large language models (LLM) not having been trained on enough data.
GenAI ends up spitting out inaccurate, even incoherent outcomes, un-contextualized to the company as an enterprise. When faced with such a difference in expectations versus reality, who wouldn’t be frustrated?
Clearly, the current status quo of data management is not working. Companies need to reimagine the way they store and access their data, and do it quickly, before they find themselves left behind.
How serious are the challenges of ready data access for organizations looking to leverage AI?
Fong: GenAI is so attractive because it is a multiplier. It promises to amplify output without the usual proportionate increase to a business’s costs and manpower. However, GenAI relies on LLMs, and LLMs are only as intelligent as the data that they are trained on.
What that means is that GenAI solutions will not be operationally useful until organizations are able to connect said solutions to the enterprise-specific data it needs to provide intelligent responses. In the same way that a car runs on fuel, GenAI runs on data – so data access is needed for GenAI to work, plain and simple.
Organizations today, however, frequently struggle with providing AI solutions with complete data, instead providing AI/MLs with departmental data sets instead. When businesses are finally able to combine multiple internal and external data sources to provide a fuller data context to AI, that is when they will achieve AI’s full potential.
Given that Gartner has predicted that 80% of businesses will adopt GenAI by 2026, it is thus crucial that organizations straighten out their data management systems, so that they can seamlessly and securely access data across multiple sources, as soon as possible. GenAI, as a trend, does not seem like it is going away any time soon.
How should legacy data infrastructure be modernized to meet the demands of AI in an organization?
Fong: The intuitive solution that most companies would turn to, is that they should now modernize their current data infrastructure. Data storage and management has seen its fair share of innovations – from mainframes to relational databases, to on-premise data warehouses to data lakes, and now, onto the cloud.
But having the latest data system addresses a symptom and not the root cause. The truth is, data infrastructure modernization will forever be a work-in-progress, because something new will always come up. Even if companies get their hands on the most cutting-edge data storage solution, it does not guarantee that all of its data will be readily accessible for GenAI’s use.
The practical realities of running a business means that, more often than not, companies end up maintaining both the latest data source as well as the legacy ones when migration runs up against issues but companies still need data from the old source to keep things running. Companies therefore need a modernizing solution that guarantees agile data access to GenAI solutions, no matter the source. Just look at banks, or airlines: they still run mainframes, on-premise databases, even as they modernize new workloads onto the cloud.
This is where virtualization technology comes in. It unifies disparate data sources onto a single layer without the need to duplicate the data or move the data onto a new system each time – just like how virtual team meeting software allows people from all around the world to come together in real-time without needing to physically travel to another location.
In this way, GenAI applications can access the data across an enterprise no matter where and how the data is stored, providing it the business-specific context needed for GenAI to run at its full potential. This approach allows for intelligent, AI-ready data delivery with governed real-time data access for business users, futureproofing the organization’s data estate.
Please share some examples of how organizations have improved GenAI efficiencies through a shift away from traditional data architecture.
Fong: At Denodo, we have enabled businesses across various sectors, such as banking & financial services, healthcare, and manufacturing, to transform their data architecture with data virtualization.
We have seen success with one of our customers, a multinational manufacturing company, who was looking to modernize its data systems in order to drive predictive analytics. What they wanted was a solution that provided democratized data its employees could self-service to use, whether for business intelligence, operations or other business functions.
The multinational manufacturing company shifted from being encumbered by a complicated, disparate data system to a virtual single-access semantic layer that allowed its workers to quickly find whatever data they need, while also having automated data governance that maintained both data security and quality. With our support, the customer is empowered to implement any GenAI-powered analytics solutions that supercharges their predictive analytics objectives, without having to worry about whether its data systems would limit the usefulness of these solutions.
When it comes to data infrastructure modernization, I firmly believe that we have to be adaptable. But adaptable in that we always rush to embrace the next change as-is? No. Adaptable in that we embrace the need for change while being practical to the realities of what it takes to change. That is how businesses can get ahead; by critically assessing what they actually need, and daring to take the steps necessary to address that need.